Assume The Random Variable X Is Normally Distributed With Mean
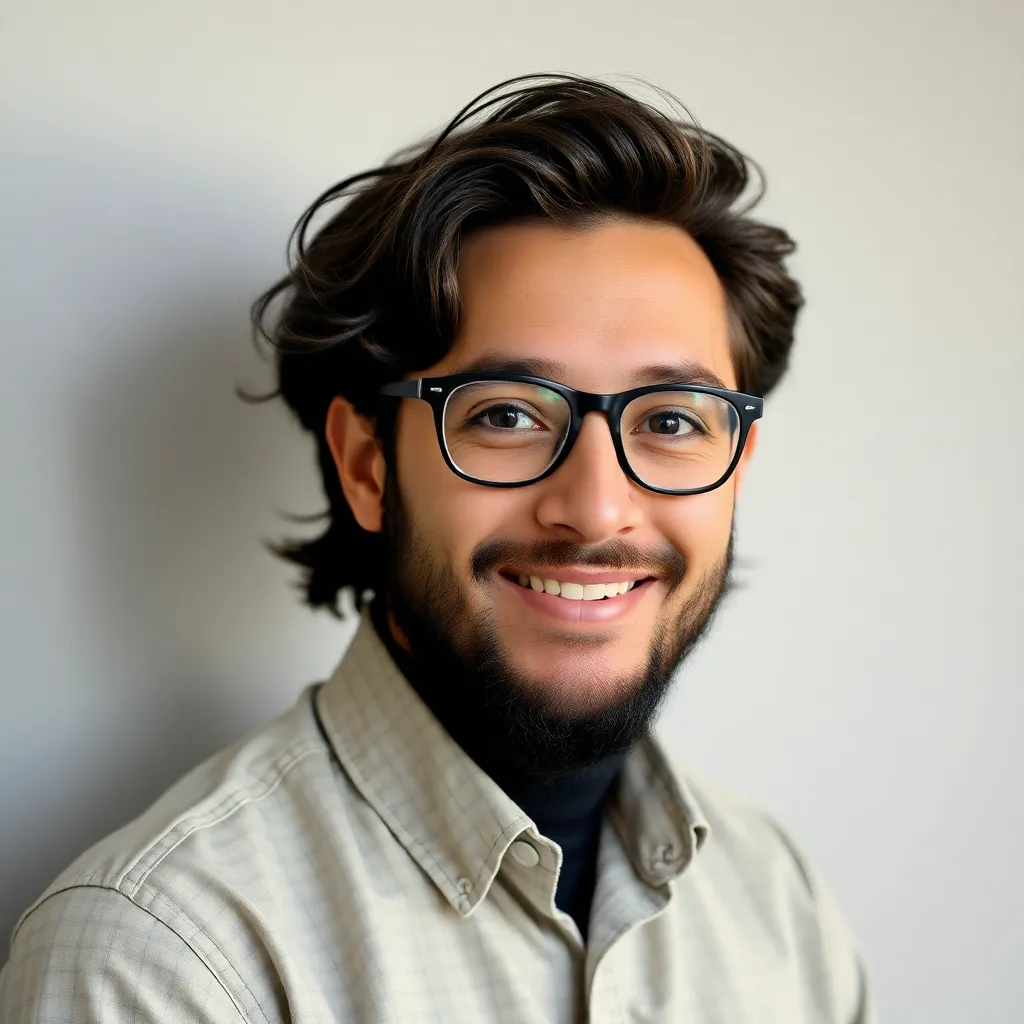
Holbox
May 10, 2025 · 6 min read
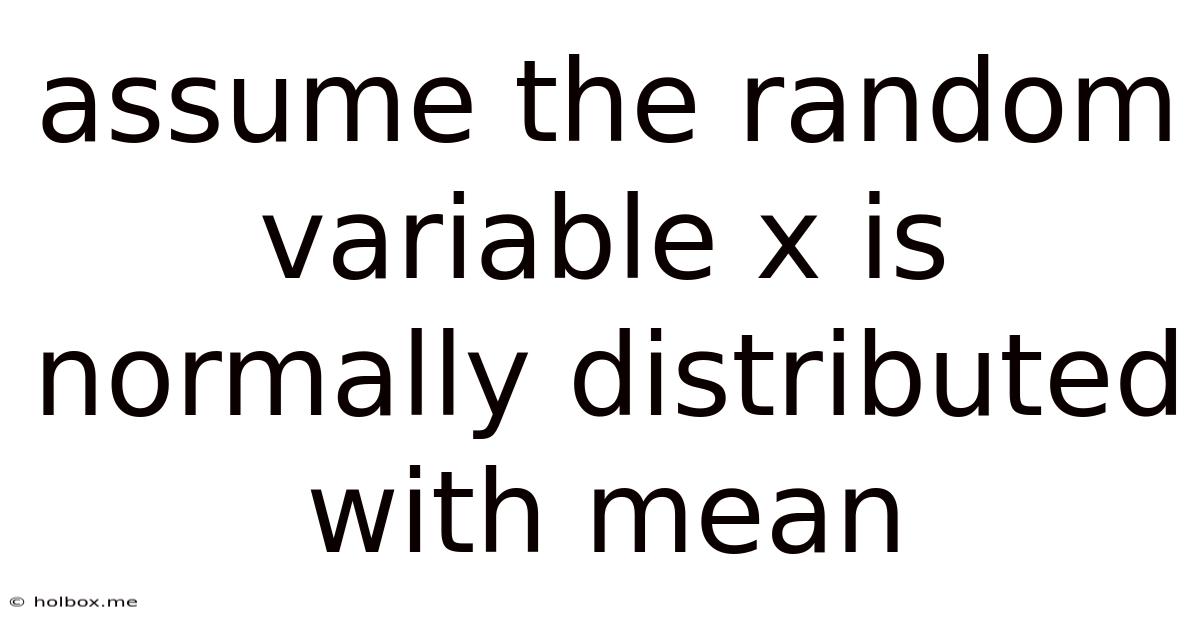
Table of Contents
- Assume The Random Variable X Is Normally Distributed With Mean
- Table of Contents
- Assume the Random Variable X is Normally Distributed with Mean: A Deep Dive into Normal Distribution
- Understanding the Normal Distribution
- Key Properties of the Normal Distribution
- Applications when X is Normally Distributed
- 1. Hypothesis Testing
- 2. Confidence Intervals
- 3. Regression Analysis
- 4. Quality Control
- 5. Modeling Natural Phenomena
- Working with Normally Distributed Data: Examples
- Dealing with Non-Normality
- Conclusion: The Power and Limitations of the Normal Distribution
- Latest Posts
- Related Post
Assume the Random Variable X is Normally Distributed with Mean: A Deep Dive into Normal Distribution
The normal distribution, also known as the Gaussian distribution, is arguably the most important probability distribution in statistics. Its ubiquity stems from the Central Limit Theorem, which states that the average of many independent and identically distributed random variables, regardless of their original distribution, tends towards a normal distribution as the number of variables increases. This makes it a powerful tool for modeling a vast array of real-world phenomena, from heights and weights to test scores and financial data. This article will explore the normal distribution in depth, focusing on scenarios where a random variable X is assumed to be normally distributed with a given mean.
Understanding the Normal Distribution
The normal distribution is characterized by its bell-shaped curve, symmetrical around its mean (μ). It's defined by two parameters:
- Mean (μ): This represents the average value of the distribution and is located at the center of the curve. It dictates the position of the curve along the x-axis.
- Standard Deviation (σ): This measures the spread or dispersion of the data around the mean. A larger standard deviation indicates a wider, flatter curve, while a smaller standard deviation results in a narrower, taller curve.
The probability density function (PDF) of the normal distribution is given by:
f(x) = (1 / (σ√(2π))) * e^(-(x-μ)^2 / (2σ^2))
While this formula may seem daunting, the key takeaway is that it precisely describes the probability of observing a value of X within a given range. The total area under the curve always equals 1, representing the total probability of all possible outcomes.
Key Properties of the Normal Distribution
Several key properties make the normal distribution so useful:
- Symmetry: The distribution is perfectly symmetrical around the mean. This means that the probability of observing a value a certain distance above the mean is equal to the probability of observing a value the same distance below the mean.
- Empirical Rule (68-95-99.7 Rule): Approximately 68% of the data falls within one standard deviation of the mean, 95% within two standard deviations, and 99.7% within three standard deviations. This rule provides a quick way to estimate probabilities without resorting to complex calculations.
- Standardization (Z-scores): Any normally distributed variable X can be standardized by converting it to a Z-score using the formula:
Z = (X - μ) / σ
. Z-scores represent the number of standard deviations a data point is away from the mean. This allows us to compare data from different normal distributions with different means and standard deviations. Z-scores follow a standard normal distribution with a mean of 0 and a standard deviation of 1. - Infinite Range: The normal distribution theoretically extends from negative infinity to positive infinity, although the probability of observing values far from the mean is extremely small.
Applications when X is Normally Distributed
The assumption that a random variable X is normally distributed is crucial in many statistical analyses. Here are some examples:
1. Hypothesis Testing
Many statistical hypothesis tests rely on the assumption of normality. For example, the t-test, used to compare the means of two groups, assumes that the data in each group is normally distributed. Violations of this assumption can affect the validity of the test results.
2. Confidence Intervals
Constructing confidence intervals for the population mean also requires the assumption of normality, especially when the sample size is small. Confidence intervals provide a range of values within which the true population mean is likely to lie with a specified level of confidence.
3. Regression Analysis
In linear regression, the assumption of normality is often made for the error terms. This assumption ensures that the estimates of the regression coefficients are unbiased and efficient.
4. Quality Control
The normal distribution is frequently used in quality control processes to monitor the variability of a manufacturing process. Control charts, which track the mean and standard deviation of a process over time, often assume that the process data is normally distributed.
5. Modeling Natural Phenomena
Many natural phenomena, such as the height and weight of individuals, follow a roughly normal distribution. This allows us to use the normal distribution to model and predict these phenomena.
Working with Normally Distributed Data: Examples
Let's illustrate with examples:
Example 1: IQ Scores
Assume that IQ scores follow a normal distribution with a mean (μ) of 100 and a standard deviation (σ) of 15. What is the probability of a randomly selected individual having an IQ score above 130?
-
Standardize: Calculate the Z-score: Z = (130 - 100) / 15 = 2
-
Consult Z-table: Look up the probability associated with Z = 2 in a standard normal distribution table (or use statistical software). This gives you the probability of a Z-score less than 2.
-
Calculate the probability above 130: Since the normal distribution is symmetrical, the probability of a Z-score greater than 2 is 1 - P(Z < 2).
Example 2: Manufacturing Process
A manufacturing process produces bolts with a mean diameter of 10 mm and a standard deviation of 0.1 mm. The process is considered to be in control if 95% of the bolts have diameters within a specified range. What is this range?
-
Use the empirical rule: 95% of the data falls within 2 standard deviations of the mean.
-
Calculate the range: The range is (μ - 2σ, μ + 2σ) = (10 - 2 * 0.1, 10 + 2 * 0.1) = (9.8 mm, 10.2 mm)
Example 3: Sampling Distribution
Suppose we are interested in the average height of adult males. We take a random sample of 100 adult males and calculate the sample mean. Even if the population distribution of heights is not perfectly normal, the Central Limit Theorem tells us that the sampling distribution of the sample mean will be approximately normal, especially with a sample size as large as 100. This allows us to make inferences about the population mean based on the sample mean.
Dealing with Non-Normality
While the normal distribution is widely applicable, it's crucial to remember that not all data follows a normal distribution. Skewed distributions, for instance, are common in many datasets. If your data deviates significantly from normality, several approaches can be taken:
- Transformations: Applying mathematical transformations to your data (e.g., logarithmic or square root transformations) can sometimes improve normality.
- Non-parametric methods: These statistical methods do not require the assumption of normality and can be used when normality cannot be reasonably assumed. Examples include the Mann-Whitney U test (non-parametric alternative to the t-test) and the Kruskal-Wallis test (non-parametric alternative to ANOVA).
- Robust methods: These statistical techniques are less sensitive to deviations from normality than traditional methods.
Conclusion: The Power and Limitations of the Normal Distribution
The normal distribution is a cornerstone of statistical inference. Its elegant mathematical properties and widespread applicability make it an indispensable tool for analyzing and interpreting data. However, it's essential to critically evaluate whether the assumption of normality is appropriate for your specific dataset. Understanding the limitations of the normal distribution and exploring alternative methods when necessary is crucial for conducting valid and reliable statistical analyses. Remember that the power of the normal distribution lies not only in its mathematical elegance but also in its ability to provide useful approximations and insights even when strict normality assumptions are not perfectly met. Using the appropriate techniques, considering the limitations and potential deviations, and employing alternative methods where necessary ensures accurate and reliable statistical analysis.
Latest Posts
Related Post
Thank you for visiting our website which covers about Assume The Random Variable X Is Normally Distributed With Mean . We hope the information provided has been useful to you. Feel free to contact us if you have any questions or need further assistance. See you next time and don't miss to bookmark.