Assume The Random Variable X Is Normally
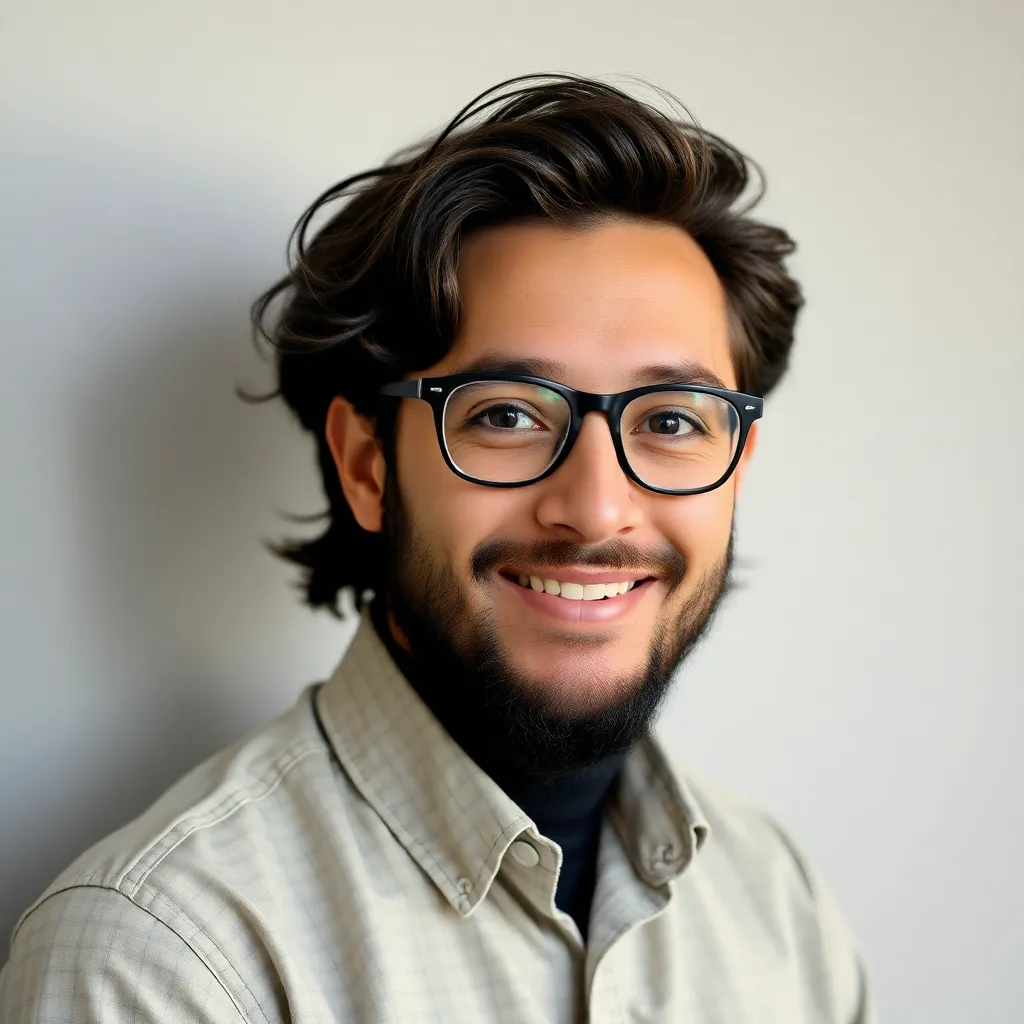
Holbox
May 11, 2025 · 7 min read
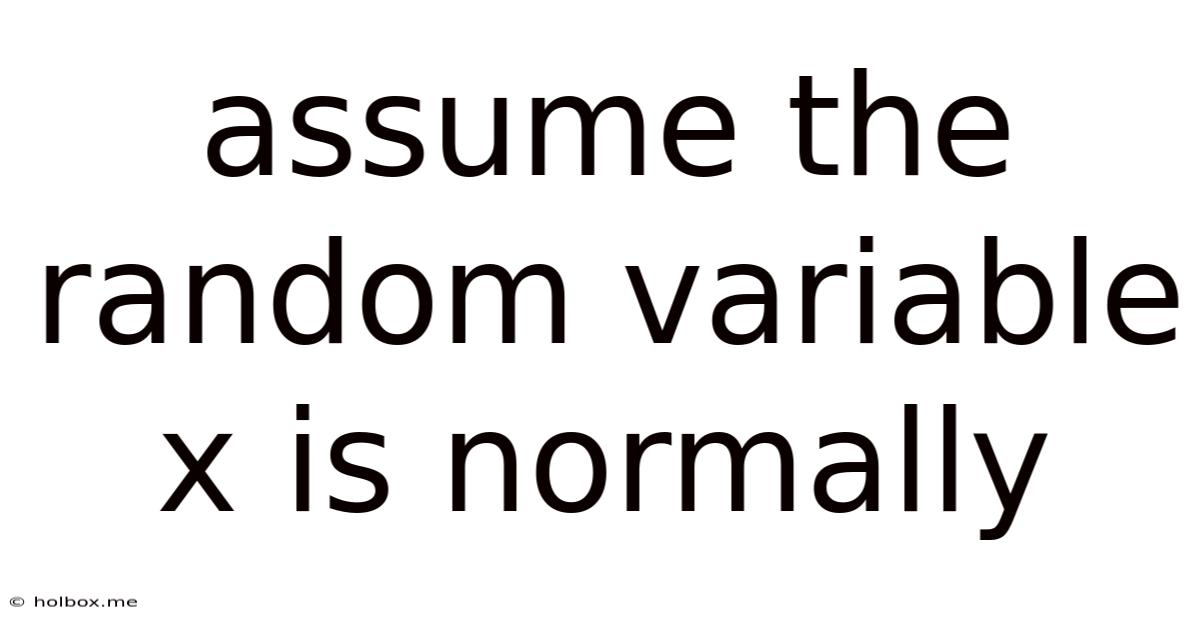
Table of Contents
- Assume The Random Variable X Is Normally
- Table of Contents
- Assume the Random Variable X is Normally Distributed: A Deep Dive into Applications and Interpretations
- Understanding the Normal Distribution
- Key Properties of a Normally Distributed Variable (X):
- The Central Limit Theorem and its Relevance
- Implications of the CLT when X is Normally Distributed:
- Applications of the Normal Distribution Assumption for X
- 1. Hypothesis Testing:
- 2. Regression Analysis:
- 3. Quality Control:
- 4. Financial Modeling:
- 5. Scientific Experiments and Data Analysis:
- Assessing Normality: Techniques and Considerations
- 1. Histograms and Q-Q Plots:
- 2. Statistical Tests:
- 3. Data Transformation:
- Dealing with Non-Normality
- 1. Use Non-parametric Methods:
- 2. Increase Sample Size:
- 3. Robust Statistical Methods:
- 4. Bootstrap Methods:
- Conclusion
- Latest Posts
- Related Post
Assume the Random Variable X is Normally Distributed: A Deep Dive into Applications and Interpretations
The normal distribution, also known as the Gaussian distribution, is a ubiquitous concept in statistics and probability. Its bell-shaped curve elegantly describes a vast range of natural phenomena, from human heights and IQ scores to measurement errors and the distribution of particle velocities in a gas. Understanding its properties and applications is crucial for anyone working with data analysis, statistical modeling, and machine learning. This comprehensive article delves into the intricacies of the normal distribution, focusing on the implications when a random variable X is assumed to be normally distributed.
Understanding the Normal Distribution
The normal distribution is characterized by two parameters: the mean (μ) and the standard deviation (σ). The mean represents the center of the distribution, while the standard deviation measures the spread or dispersion of the data around the mean. A higher standard deviation indicates greater variability. The probability density function (PDF) of a normal distribution is given by:
f(x) = (1/σ√(2π)) * e^(-(x-μ)²/(2σ²))
This seemingly complex formula encapsulates the bell-shaped curve's elegant properties. The curve is symmetrical around the mean, meaning the probabilities of observing values above and below the mean are equal. Furthermore, approximately 68% of the data falls within one standard deviation of the mean, 95% within two standard deviations, and 99.7% within three standard deviations – this is often referred to as the empirical rule or the 68-95-99.7 rule.
Key Properties of a Normally Distributed Variable (X):
- Symmetry: The distribution is perfectly symmetrical around its mean (μ).
- Mean, Median, and Mode: The mean, median, and mode are all equal to μ.
- Standard Deviation: σ determines the spread of the distribution. A larger σ implies greater variability.
- Area Under the Curve: The total area under the curve is equal to 1, representing the total probability.
- Infinite Range: The normal distribution extends infinitely in both directions along the x-axis, although the probability density becomes extremely small far from the mean.
The Central Limit Theorem and its Relevance
The Central Limit Theorem (CLT) is a cornerstone of statistical inference. It states that the distribution of the sample means from a large number of independent random samples, regardless of the original population's distribution, will approximate a normal distribution as the sample size increases. This is a powerful result because it allows us to make inferences about the population mean even when we don't know the underlying population distribution. If X is normally distributed, then the CLT's impact is amplified, leading to more accurate and reliable estimations.
Implications of the CLT when X is Normally Distributed:
- Faster Convergence: When the underlying population is already normally distributed, the convergence to a normal distribution of sample means happens much faster than when the underlying distribution is non-normal.
- Smaller Sample Sizes: Accurate inferences about the population mean can be achieved with smaller sample sizes compared to situations where the underlying population distribution isn't normal.
- Improved Accuracy of Confidence Intervals: Confidence intervals, which provide a range of values likely to contain the true population mean, are more precise and reliable when X is normally distributed.
Applications of the Normal Distribution Assumption for X
The assumption that a random variable X is normally distributed underpins a wide range of statistical techniques and applications. Let's explore some key areas:
1. Hypothesis Testing:
Many statistical hypothesis tests rely on the assumption of normality. For example, the t-test, used to compare the means of two groups, assumes that the data in each group is normally distributed. Similarly, ANOVA (Analysis of Variance), used to compare the means of three or more groups, also relies on the normality assumption. If X is normally distributed, the results of these tests are more robust and reliable.
2. Regression Analysis:
In linear regression, the assumption of normally distributed errors (residuals) is crucial for the validity of the statistical inferences made. If the errors aren't normally distributed, the estimated regression coefficients may be biased, and the p-values associated with the coefficients might be unreliable. A normally distributed X, particularly when it's an independent variable, can contribute to a more accurate model.
3. Quality Control:
In quality control, the normal distribution is used to model the variability of a manufacturing process. By assuming that the measurements of a particular quality characteristic are normally distributed, we can set control limits and monitor the process to ensure that it remains stable and produces products within acceptable tolerances. This proactive approach minimizes defects and maintains product quality.
4. Financial Modeling:
The normal distribution plays a significant role in financial modeling, particularly in assessing risk. For instance, asset returns are often modeled as normally distributed, which allows us to calculate probabilities of different outcomes and make informed investment decisions. However, it's crucial to note that this assumption is often debated due to the occurrence of "fat tails" in real financial data, which represents more extreme events than predicted by the normal distribution.
5. Scientific Experiments and Data Analysis:
Numerous scientific fields, ranging from biology and medicine to physics and engineering, rely on the normal distribution. Experimental data often conforms to a normal distribution after collecting data from a sufficiently large sample size (as described by the CLT). Analyzing data under the assumption of normality helps to extract meaningful insights and make informed conclusions.
Assessing Normality: Techniques and Considerations
Before assuming that X is normally distributed, it's crucial to assess whether this assumption is justified. Several techniques can help determine the normality of a dataset:
1. Histograms and Q-Q Plots:
Histograms provide a visual representation of the data's distribution. A bell-shaped histogram suggests normality. Q-Q plots (Quantile-Quantile plots) compare the quantiles of the data to the quantiles of a normal distribution. If the data is normally distributed, the points in the Q-Q plot will fall approximately along a straight line.
2. Statistical Tests:
Several statistical tests, such as the Shapiro-Wilk test and the Kolmogorov-Smirnov test, can formally assess the normality of a dataset. These tests provide a p-value, which indicates the probability of observing the data if it were truly normally distributed. A small p-value (typically less than 0.05) suggests that the data is not normally distributed.
3. Data Transformation:
If the data is not normally distributed, transformations like logarithmic or square root transformations can sometimes make it more normally distributed. These transformations can stabilize variance and improve the symmetry of the data.
Dealing with Non-Normality
If the assessment reveals that X is not normally distributed, several strategies can be employed:
1. Use Non-parametric Methods:
Non-parametric methods are statistical techniques that don't rely on the assumption of normality. Examples include the Mann-Whitney U test (a non-parametric alternative to the t-test) and the Kruskal-Wallis test (a non-parametric alternative to ANOVA).
2. Increase Sample Size:
As the sample size increases, the CLT becomes more relevant, and the distribution of sample means approaches normality, even if the underlying population isn't normally distributed. This allows the use of parametric methods.
3. Robust Statistical Methods:
Robust statistical methods are less sensitive to deviations from normality. These methods are designed to perform well even when the data contains outliers or deviates from the normality assumption.
4. Bootstrap Methods:
Bootstrap methods are resampling techniques that can be used to estimate the sampling distribution of statistics without relying on the assumption of normality. This approach is particularly useful when dealing with small sample sizes or non-normal data.
Conclusion
The assumption that a random variable X is normally distributed is a powerful tool in statistical analysis. It underlies many widely used statistical methods and provides a framework for modeling and interpreting data. However, it's crucial to carefully assess the normality of the data before making this assumption. By utilizing appropriate normality tests, visualizations, and considering alternative approaches when necessary, researchers and analysts can ensure the robustness and validity of their statistical conclusions, whether X is normally distributed or not. This careful consideration ensures the accuracy and reliability of any resulting inferences and ultimately contributes to a more rigorous and scientifically sound understanding of the data at hand. Remember that the goal is to accurately represent the data and draw meaningful conclusions, adapting techniques as needed based on the data's characteristics.
Latest Posts
Related Post
Thank you for visiting our website which covers about Assume The Random Variable X Is Normally . We hope the information provided has been useful to you. Feel free to contact us if you have any questions or need further assistance. See you next time and don't miss to bookmark.