Ap Stats Unit 7 Progress Check Mcq Part C
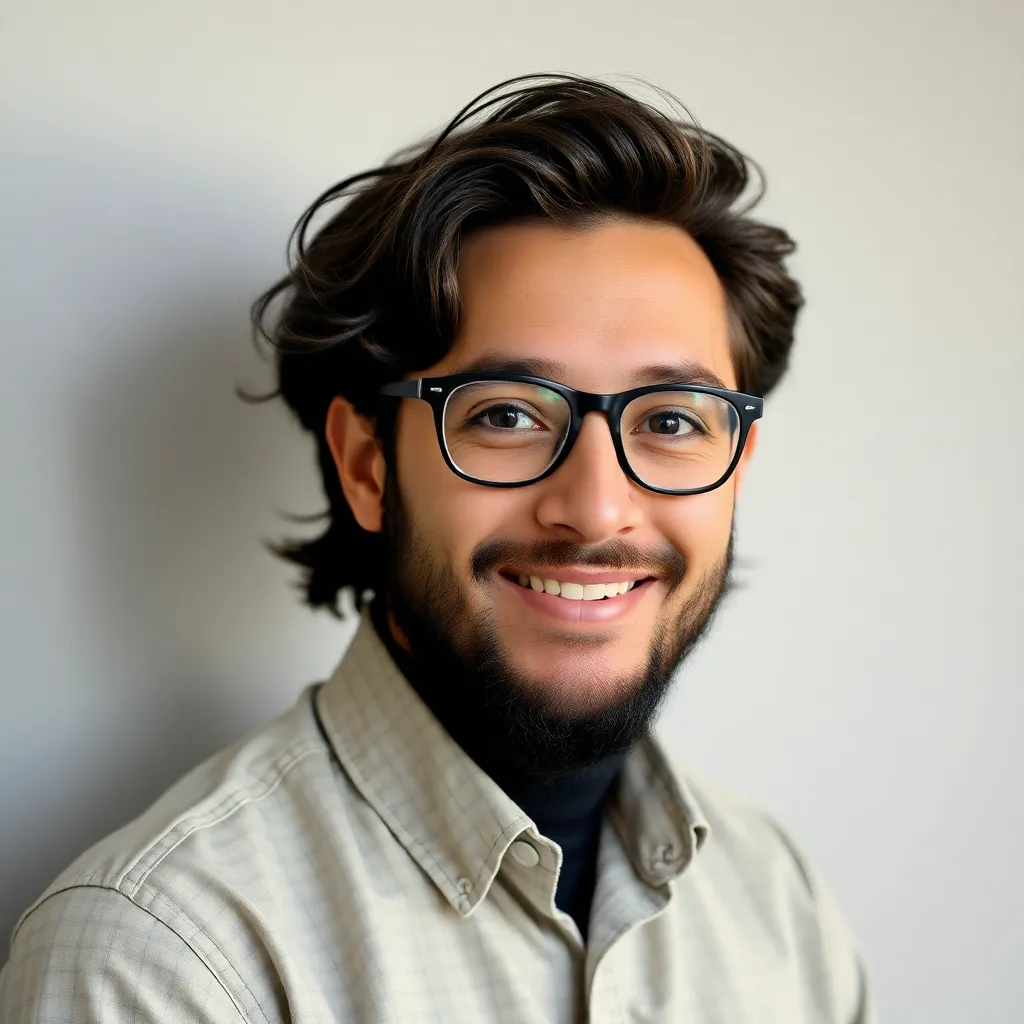
Holbox
May 07, 2025 ยท 7 min read
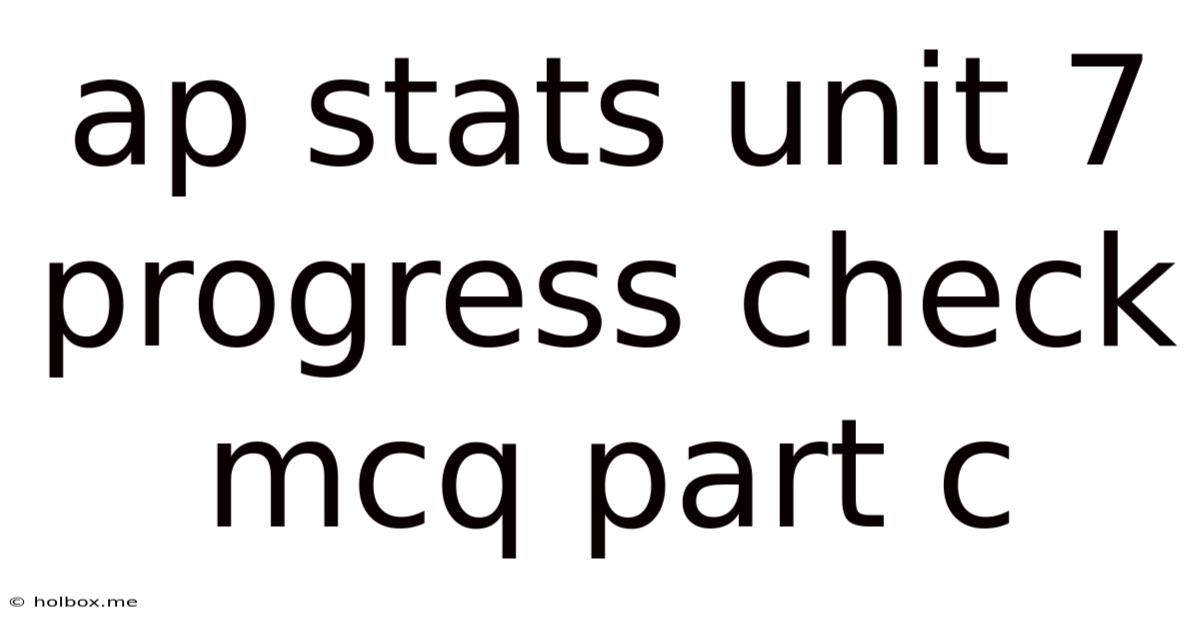
Table of Contents
- Ap Stats Unit 7 Progress Check Mcq Part C
- Table of Contents
- AP Stats Unit 7 Progress Check: MCQ Part C โ A Deep Dive
- Understanding the Core Concepts of Unit 7
- 1. Sampling Distributions: The Foundation of Inference
- 2. Confidence Intervals for Proportions
- 3. Hypothesis Testing for Proportions
- Tackling AP Stats Unit 7 Progress Check MCQ Part C
- 1. Interpreting Sampling Distribution Graphs
- 2. Combining Confidence Intervals and Hypothesis Tests
- 3. Complex Scenarios and Data Analysis
- 4. Understanding Type I and Type II Errors
- 5. Applying the Central Limit Theorem
- Strategies for Success on Part C
- Latest Posts
- Related Post
AP Stats Unit 7 Progress Check: MCQ Part C โ A Deep Dive
Unit 7 of AP Statistics, focusing on sampling distributions and inference for proportions, is a crucial stepping stone towards mastering the subject. The Progress Check MCQs, particularly Part C, often present challenging questions that require a solid understanding of concepts and their application. This comprehensive guide will dissect the key topics covered in Unit 7, focusing on the intricacies of Part C multiple-choice questions, equipping you with the strategies and knowledge needed to excel.
Understanding the Core Concepts of Unit 7
Before tackling the complexities of the Progress Check, let's solidify our understanding of the fundamental concepts:
1. Sampling Distributions: The Foundation of Inference
The heart of Unit 7 lies in understanding sampling distributions. A sampling distribution is the probability distribution of a statistic (like the sample proportion, ๐ฬ) obtained from a large number of samples drawn from the same population. It's crucial to grasp that this isn't the distribution of the population itself, but rather the distribution of possible sample statistics. This distinction is vital for accurate inference.
Key Characteristics:
- Center: The mean of the sampling distribution of ๐ฬ is equal to the population proportion, p.
- Spread: The standard deviation of the sampling distribution of ๐ฬ (also called the standard error, SE) is given by โ[p(1-p)/n], where 'n' is the sample size. Note that a larger sample size leads to a smaller standard error, indicating less variability in the sample proportions.
- Shape: For sufficiently large sample sizes (typically when np โฅ 10 and n(1-p) โฅ 10), the sampling distribution of ๐ฬ is approximately normal, thanks to the Central Limit Theorem. This normality is crucial for conducting hypothesis tests and constructing confidence intervals.
2. Confidence Intervals for Proportions
A confidence interval provides a range of plausible values for a population parameter (in this case, the population proportion, p). A 95% confidence interval, for instance, means that if we were to repeatedly sample and construct confidence intervals, 95% of those intervals would contain the true population proportion.
The formula for a confidence interval for a population proportion is:
๐ฬ ยฑ z*โ[๐ฬ(1-๐ฬ)/n]
Where:
- ๐ฬ is the sample proportion
- z* is the critical z-score corresponding to the desired confidence level (e.g., 1.96 for a 95% confidence interval)
- n is the sample size
Interpreting Confidence Intervals: It's crucial to understand the correct interpretation. A 95% confidence interval does not mean there's a 95% chance the true proportion lies within the interval. The true proportion is either in the interval or it isn't; the 95% refers to the procedure's reliability in generating intervals that capture the true value.
3. Hypothesis Testing for Proportions
Hypothesis testing allows us to formally test claims about a population proportion. We set up null and alternative hypotheses, calculate a test statistic (often a z-statistic), and determine a p-value to assess the strength of evidence against the null hypothesis.
Steps involved:
- State Hypotheses: Define the null hypothesis (Hโ) โ a statement of no effect โ and the alternative hypothesis (Hโ) โ the claim we're testing.
- Check Conditions: Verify that the conditions for inference are met (random sample, 10% condition, success/failure condition).
- Calculate Test Statistic: Compute the z-statistic: (๐ฬ - pโ) / โ[pโ(1-pโ)/n], where pโ is the proportion specified in the null hypothesis.
- Find p-value: Determine the probability of observing a sample proportion as extreme as, or more extreme than, the one obtained, assuming the null hypothesis is true.
- Make a Decision: Compare the p-value to the significance level (ฮฑ, often 0.05). If the p-value is less than ฮฑ, we reject the null hypothesis; otherwise, we fail to reject it.
Tackling AP Stats Unit 7 Progress Check MCQ Part C
Part C questions often integrate multiple concepts from Unit 7, requiring a deeper understanding and problem-solving skills. They frequently involve:
1. Interpreting Sampling Distribution Graphs
Expect questions showing graphs of sampling distributions. You need to be able to interpret the center (representing the population proportion), the spread (reflecting the standard error), and the shape (assessing whether the normal approximation is valid). Questions might ask about the impact of changing the sample size or the population proportion on the graph.
Example: A graph depicts the sampling distribution of ๐ฬ for various sample sizes. The question might ask how the standard error changes as the sample size increases. Understanding that a larger sample size leads to a smaller standard error is crucial here.
2. Combining Confidence Intervals and Hypothesis Tests
Part C might present a scenario where you're given a confidence interval and asked to determine whether a particular hypothesis would be rejected at a certain significance level. This requires a strong understanding of the relationship between confidence intervals and hypothesis tests. If the value specified in the null hypothesis falls outside the confidence interval, it suggests that the null hypothesis would likely be rejected.
Example: A 95% confidence interval for a population proportion is (0.45, 0.55). A question might ask whether the null hypothesis Hโ: p = 0.6 would be rejected at a 0.05 significance level. Since 0.6 is outside the interval, the null hypothesis would be rejected.
3. Complex Scenarios and Data Analysis
Expect questions involving more realistic scenarios with potentially confounding factors. You might be given data from a survey and asked to construct a confidence interval or perform a hypothesis test, requiring careful attention to detail and a clear understanding of the context. These questions often test your ability to identify potential biases or limitations in the sampling method.
Example: A question might describe a survey about political preferences with a potential sampling bias, asking you to explain how this bias might affect the validity of any inferences drawn from the data.
4. Understanding Type I and Type II Errors
Part C frequently tests your understanding of Type I and Type II errors. A Type I error occurs when you reject the null hypothesis when it's actually true, while a Type II error occurs when you fail to reject the null hypothesis when it's false. Understanding the implications of these errors and how to minimize them is vital.
Example: A question might present a scenario and ask you to identify the consequences of making a Type I or Type II error in that context. This requires careful consideration of the real-world implications of the decision made based on the hypothesis test.
5. Applying the Central Limit Theorem
The Central Limit Theorem (CLT) is fundamental. Many questions will hinge on your ability to apply the CLT, especially when dealing with sample proportions. Remember that the CLT states that the sampling distribution of the sample mean (or proportion) becomes approximately normal as the sample size increases, regardless of the shape of the original population distribution.
Example: A question might involve a non-normal population distribution but ask about the sampling distribution of the sample proportion for a large sample size. Correctly applying the CLT would require understanding that even though the population is not normal, the sampling distribution of ๐ฬ will be approximately normal because of the large sample size.
Strategies for Success on Part C
To conquer the challenges of Part C, adopt these strategies:
- Master the Fundamentals: A thorough understanding of sampling distributions, confidence intervals, and hypothesis testing is paramount.
- Practice, Practice, Practice: Work through numerous practice problems, focusing on complex scenarios that integrate multiple concepts.
- Visualize: Sketching graphs of sampling distributions can greatly aid in understanding the concepts and solving problems.
- Understand the Context: Pay close attention to the specific context of each problem. This helps identify potential biases or limitations.
- Review Formulas: Familiarize yourself with the key formulas for confidence intervals and hypothesis tests.
- Analyze Carefully: Break down complex problems into smaller, manageable steps.
By diligently applying these strategies and mastering the core concepts, you will significantly improve your performance on the AP Stats Unit 7 Progress Check MCQ Part C, setting you up for success on the AP exam. Remember, consistent practice and a deep conceptual understanding are the keys to unlocking success in statistics.
Latest Posts
Related Post
Thank you for visiting our website which covers about Ap Stats Unit 7 Progress Check Mcq Part C . We hope the information provided has been useful to you. Feel free to contact us if you have any questions or need further assistance. See you next time and don't miss to bookmark.