All Of The Following Are Examples Of Cross-sectional Data Except:
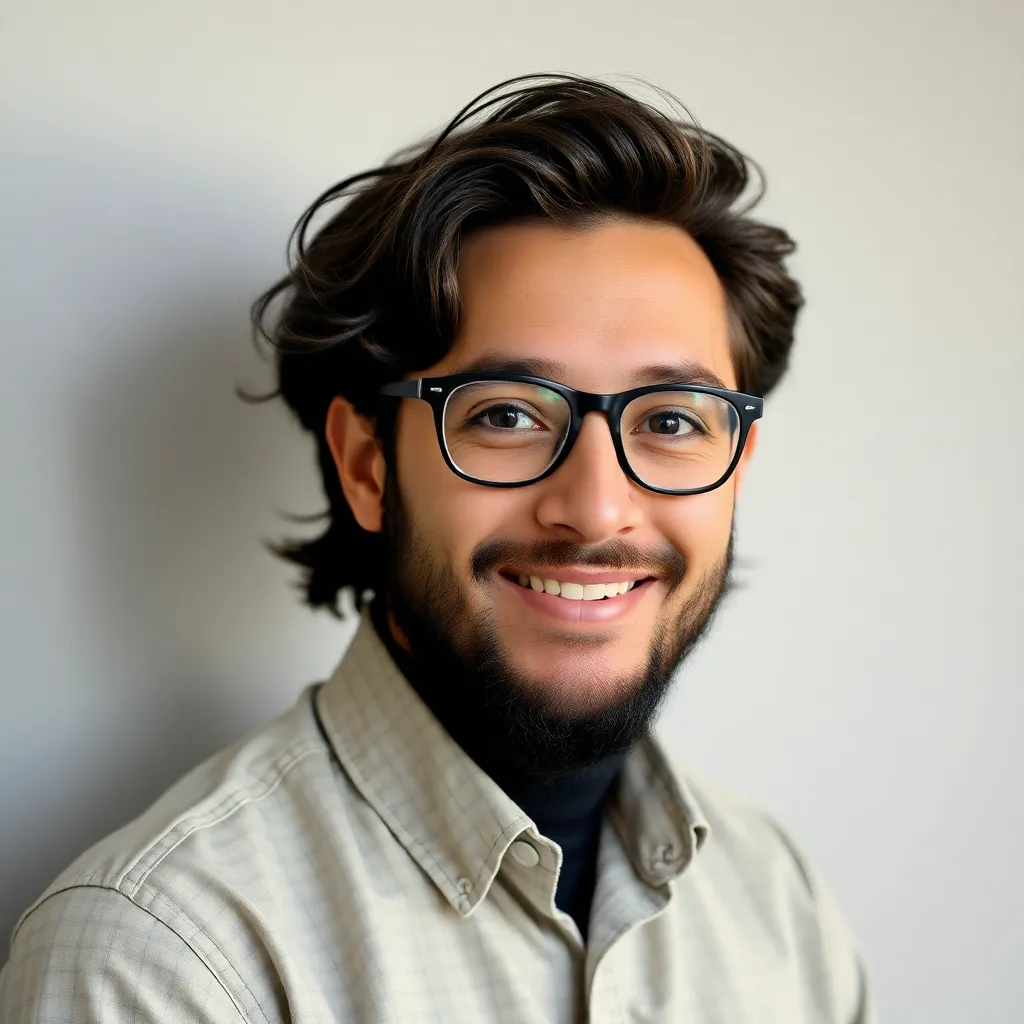
Holbox
May 11, 2025 · 6 min read
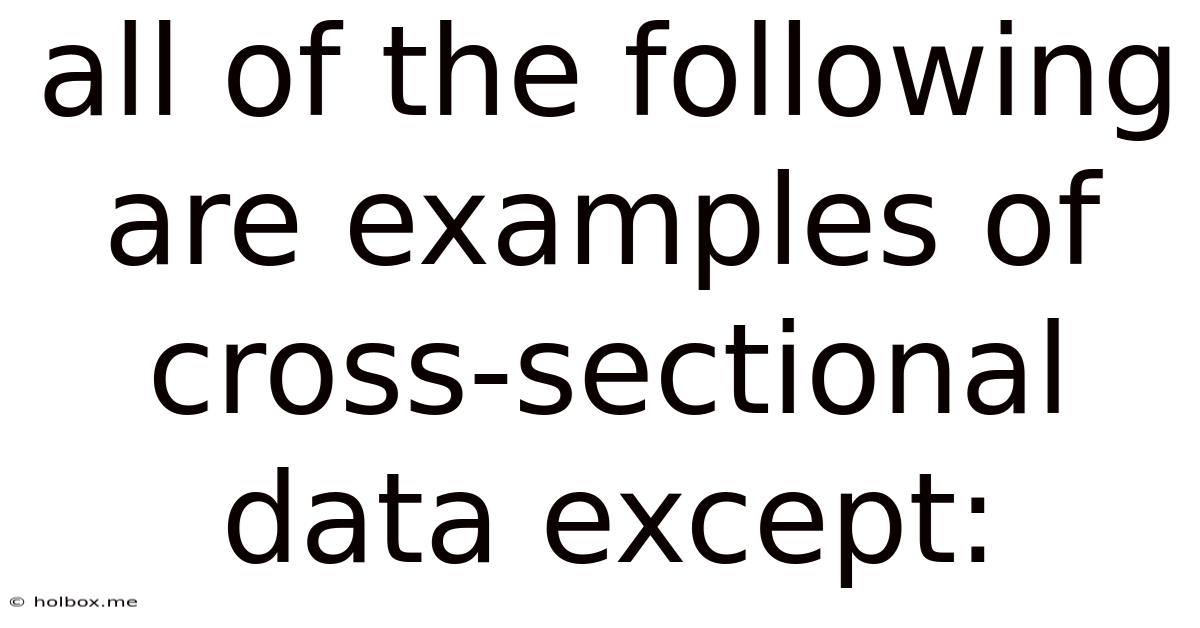
Table of Contents
- All Of The Following Are Examples Of Cross-sectional Data Except:
- Table of Contents
- All of the Following Are Examples of Cross-Sectional Data Except: Understanding Different Data Types in Research
- Defining Cross-Sectional Data
- Key Characteristics of Cross-Sectional Data:
- Examples of Cross-Sectional Data:
- 1. A Survey of Consumer Preferences:
- 2. A Census:
- 3. A Study of Healthcare Utilization:
- 4. Economic Data for a Single Year:
- 5. A Study of Student Performance:
- Distinguishing Cross-Sectional Data from Other Data Types:
- 1. Longitudinal Data:
- 2. Time-Series Data:
- What is NOT Cross-Sectional Data?
- Advantages and Limitations of Cross-Sectional Data:
- Advantages:
- Limitations:
- Conclusion:
- Latest Posts
- Latest Posts
- Related Post
All of the Following Are Examples of Cross-Sectional Data Except: Understanding Different Data Types in Research
Cross-sectional data is a type of data collected by observing many subjects (such as individuals, firms, countries, or regions) at the same point of time, or without regard to differences in time. Understanding the nuances of cross-sectional data is crucial for researchers across various fields, from economics and sociology to epidemiology and marketing. This article delves deep into the definition and characteristics of cross-sectional data, provides clear examples, and highlights the key difference that distinguishes it from other data types. By the end, you will be able to confidently identify cross-sectional data and differentiate it from longitudinal and time-series data.
Defining Cross-Sectional Data
Cross-sectional data offers a snapshot of a population at a specific moment. It captures information on various characteristics of the subjects at that single point in time. Think of it like taking a photograph – it freezes a moment in time, providing a record of what existed at that precise instant. This contrasts sharply with other data collection methods that track changes over time.
Key Characteristics of Cross-Sectional Data:
- Single Point in Time: The most defining characteristic. Data is gathered at one specific time period.
- Multiple Subjects: It involves observations from a large number of subjects, allowing for analysis of relationships between different variables.
- Snapshot of a Population: Provides a static picture of the population's characteristics at a given point.
- Descriptive and Correlational: Primarily useful for descriptive statistics and exploring correlations between variables. Establishing causality is more challenging.
Examples of Cross-Sectional Data:
Let's illustrate with concrete examples to solidify your understanding. The following are all prime examples of cross-sectional data:
1. A Survey of Consumer Preferences:
Imagine a market research firm conducting a survey to understand consumer preferences for a new product. They collect data from 1000 respondents at a single point in time, asking questions about demographics, brand awareness, purchasing habits, and opinions about the new product. This constitutes cross-sectional data because the data is gathered from a large sample at one particular moment.
2. A Census:
National censuses provide a classic example. Governments conduct censuses to gather demographic information about their population – age, gender, income, education, occupation – at a specific point in time. The snapshot of the population's characteristics at that moment constitutes cross-sectional data.
3. A Study of Healthcare Utilization:
Researchers might study healthcare utilization patterns by collecting data from hospital records on patient demographics, diagnoses, treatments, and length of stay for a specific month. This one-time data collection on many patients represents cross-sectional data.
4. Economic Data for a Single Year:
Collecting data on GDP, inflation, unemployment rates, and other economic indicators for a single year provides cross-sectional data for that particular year. It gives a picture of the economy's state at that specific point in time.
5. A Study of Student Performance:
Examining the academic performance of students (GPA, test scores) within a single semester at a university generates cross-sectional data. It captures the academic standing of many students at a particular time.
Distinguishing Cross-Sectional Data from Other Data Types:
The key to understanding cross-sectional data lies in distinguishing it from longitudinal and time-series data. Let’s explore these distinctions:
1. Longitudinal Data:
Longitudinal data involves observing the same subjects over a period of time. This allows researchers to study changes in individuals or groups over time. A longitudinal study could follow the same group of children from birth to adulthood, tracking their physical development, cognitive abilities, and social behavior. This is fundamentally different from cross-sectional data, which focuses on a single point in time.
Key Difference: Longitudinal studies track changes over time within the same subjects, while cross-sectional studies examine multiple subjects at a single point in time.
2. Time-Series Data:
Time-series data tracks a single subject (e.g., stock price, temperature, sales figures) over time. It’s a sequence of data points collected at successive time intervals. For instance, charting the daily closing price of a stock over a year would generate time-series data.
Key Difference: Time-series data focuses on a single subject over time, whereas cross-sectional data examines many subjects at a single point in time.
What is NOT Cross-Sectional Data?
Now, let's address the core question: All of the following are examples of cross-sectional data EXCEPT: The answer depends on the options presented. However, any dataset that involves repeated measurements of the same subjects over time would NOT be cross-sectional. This includes:
- Panel Data: Panel data is a combination of cross-sectional and time-series data. It tracks multiple subjects over time. For example, a panel dataset might track the sales of several different companies over a five-year period.
- Repeated Measures Data: Similar to panel data, repeated measures data involves multiple observations of the same subjects at different times. This is frequently used in experiments to track the effect of an intervention.
- Cohort Studies: These studies follow a specific group of individuals (a cohort) over time. While they may gather data at multiple points, the focus remains on tracking the same group, making it different from cross-sectional data.
Examples of options that would not be cross-sectional data in a multiple-choice question:
- Monthly sales figures for a single store over a year. (Time-series data)
- Weight measurements of the same group of individuals taken every six months for two years. (Longitudinal data)
- Annual GDP data from 1990-2023. (Time-series data)
- Tracking the growth of a specific plant every day for a month. (Time-series data)
- Changes in patient blood pressure over a course of treatment. (Longitudinal data)
Advantages and Limitations of Cross-Sectional Data:
Advantages:
- Cost-Effective: Generally less expensive and time-consuming to collect than longitudinal data.
- Large Sample Sizes: It's feasible to collect data from a large number of subjects, leading to more robust statistical analysis.
- Easy to Interpret: The data is relatively straightforward to interpret and analyze, requiring less sophisticated statistical methods.
- Provides a Broad Overview: Gives a broad snapshot of the population at a specific moment, useful for descriptive analysis and understanding prevalence.
Limitations:
- Cannot Establish Causality: Difficult to establish causal relationships between variables due to the lack of time dimension. Correlations observed may not represent actual causal links.
- Limited Information about Change: Doesn't provide information about changes over time. Trends and dynamic processes are not captured.
- Susceptible to Cohort Effects: Variations in the data might reflect differences between cohorts rather than true changes over time.
- Snapshot may not be Representative: The snapshot captured may not be representative of the population at other times, particularly during periods of rapid change.
Conclusion:
Cross-sectional data provides a valuable snapshot of a population at a given moment, offering insights into relationships between variables. However, it's crucial to understand its limitations, particularly the inability to infer causality and track changes over time. By contrasting cross-sectional data with longitudinal and time-series data, researchers can choose the appropriate data collection method to address their specific research questions. Mastering the ability to distinguish these data types is a cornerstone of effective research design and analysis. Remember that the key differentiator for cross-sectional data is the single point in time data collection across many subjects. Understanding this fundamental characteristic will empower you to effectively analyze and interpret data in your chosen field.
Latest Posts
Latest Posts
-
How Many Cups Is 45 Ml
May 19, 2025
-
What Is 45 Mph In Km
May 19, 2025
-
How Many Pounds In 7 Kg
May 19, 2025
-
How Many Weeks Is 18 Days
May 19, 2025
-
How Many Ml Is 10 5 Oz
May 19, 2025
Related Post
Thank you for visiting our website which covers about All Of The Following Are Examples Of Cross-sectional Data Except: . We hope the information provided has been useful to you. Feel free to contact us if you have any questions or need further assistance. See you next time and don't miss to bookmark.