A Small Business Owner Has Created A Linear Regression
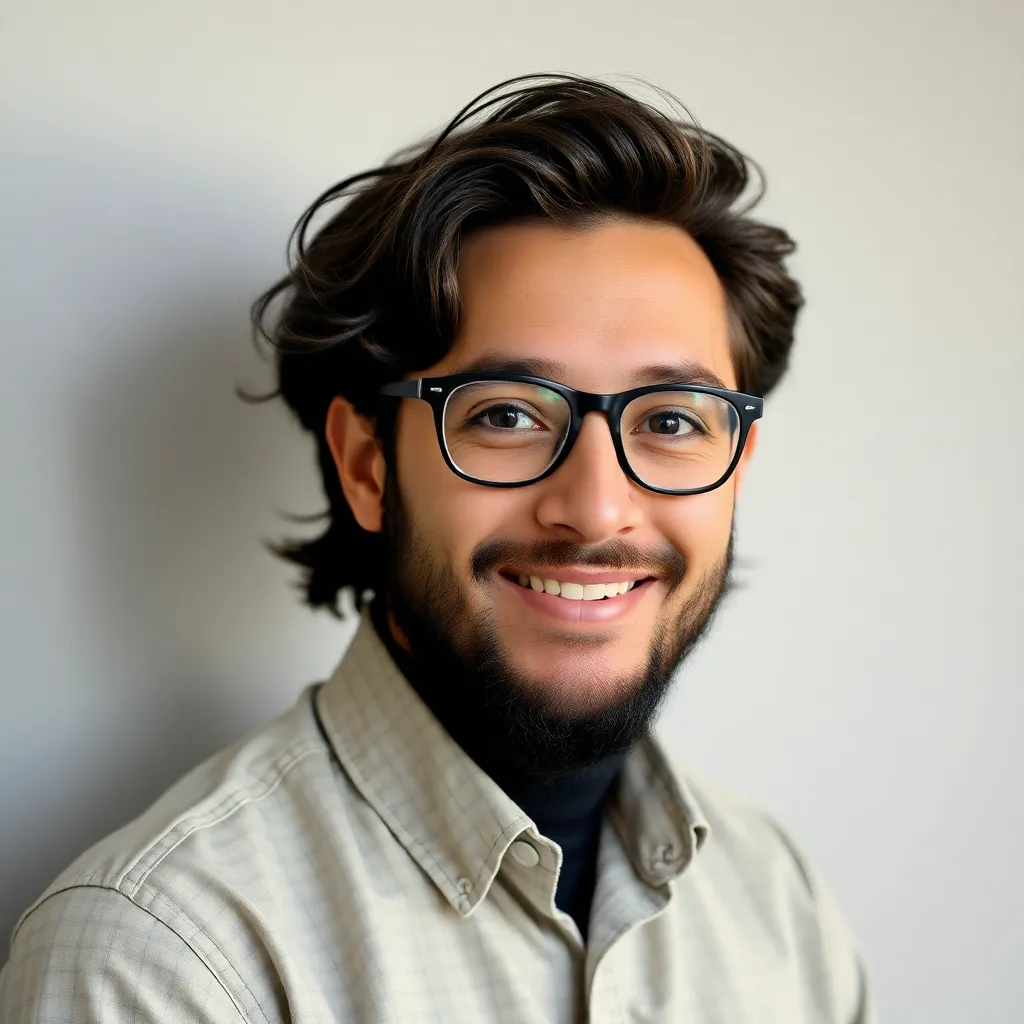
Holbox
May 09, 2025 · 5 min read
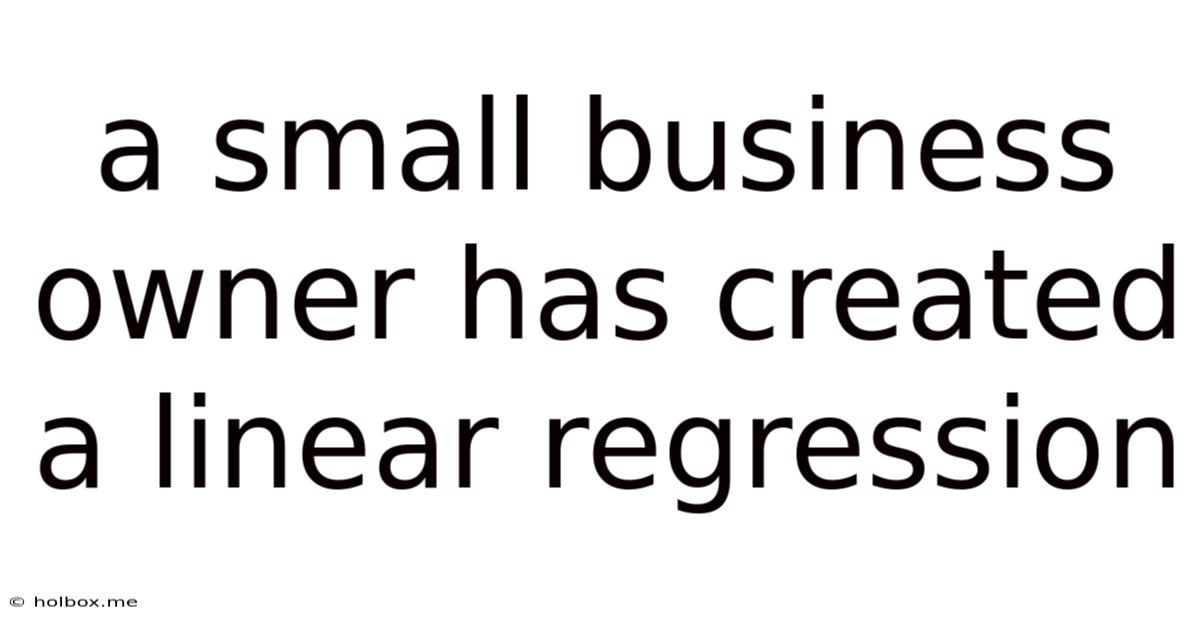
Table of Contents
- A Small Business Owner Has Created A Linear Regression
- Table of Contents
- A Small Business Owner's Journey into Linear Regression: Predicting Success One Equation at a Time
- Sarah's Story: From Intuition to Insight
- Understanding Linear Regression: A Simple Explanation
- The Data Gathering Phase: The Foundation of Success
- Building the Regression Model: Choosing the Right Tools
- Interpreting the Results: Unveiling Valuable Insights
- Refining the Model: Iteration and Improvement
- Using the Model for Decision Making: Practical Applications
- Beyond Linear Regression: Expanding the Data Analysis Toolkit
- Conclusion: Embracing Data for Small Business Success
- Latest Posts
- Latest Posts
- Related Post
A Small Business Owner's Journey into Linear Regression: Predicting Success One Equation at a Time
For many small business owners, success feels like navigating a fog. You're working hard, but predicting future sales, optimizing pricing, or understanding the impact of marketing campaigns can feel like guessing games. This is where the power of linear regression comes in. While it might sound intimidating, linear regression is a surprisingly accessible tool that can transform how a small business owner makes decisions. This article will explore how a hypothetical small business owner, let's call her Sarah, used linear regression to gain valuable insights and improve her business.
Sarah's Story: From Intuition to Insight
Sarah owns a charming bakery, "Sweet Surrender," specializing in artisan breads and pastries. She's passionate about her craft, but her business decisions were largely based on intuition and gut feelings. While her bakery enjoyed steady growth, Sarah felt she could achieve more with a more data-driven approach. She decided to explore the world of data analysis, starting with a simple yet powerful technique: linear regression.
Understanding Linear Regression: A Simple Explanation
At its core, linear regression is a statistical method used to model the relationship between a dependent variable (what you're trying to predict) and one or more independent variables (factors that influence the dependent variable). Imagine it as finding the best-fitting straight line through a scatter plot of data points. The equation of this line represents the relationship, allowing you to predict the dependent variable's value given the independent variables.
For Sarah, her dependent variable was her monthly sales. She considered several independent variables that might influence her sales:
- Price: The average price of her products.
- Advertising Spend: The amount she spent on local advertising.
- Seasonal Factors: Dummy variables representing different seasons (e.g., 1 for summer, 0 for other seasons).
- Number of Events: The number of local events held in her area.
The Data Gathering Phase: The Foundation of Success
Before Sarah could apply linear regression, she needed data. She meticulously collected data for the past two years, diligently recording her monthly sales and the values of her chosen independent variables. This careful data collection is crucial – garbage in, garbage out, as they say. Inaccurate or incomplete data would lead to unreliable results.
Pro Tip: Consider using spreadsheets or dedicated database software to store and manage your data. This will make data analysis significantly easier.
Building the Regression Model: Choosing the Right Tools
Sarah, lacking a background in advanced statistics, chose a user-friendly statistical software package (many free and paid options exist). She entered her data, selecting "linear regression" as her analysis method. The software then performed the calculations to find the best-fitting line and provide the regression equation.
The equation she obtained might look something like this:
Sales = 5000 + 100 * Price + 5 * Advertising Spend + 1500 * Summer + 800 * Number of Events
This equation indicates the relationship between her monthly sales and the selected independent variables. For instance, a $1 increase in average price is associated with a $100 increase in sales, while an extra $1 spent on advertising results in a $5 increase in sales.
Important Note: The specific coefficients (the numbers multiplying the variables) depend on the data. They represent the estimated effect of each independent variable on sales.
Interpreting the Results: Unveiling Valuable Insights
The regression analysis revealed several key insights for Sarah:
- Price Elasticity: The positive coefficient for "Price" suggested that increasing prices, within reason, might positively impact her revenue. This was unexpected, possibly due to the perception of higher quality associated with higher prices for her artisan products.
- Advertising ROI: The positive coefficient for "Advertising Spend" confirmed the effectiveness of her local advertising campaigns, though with a modest return.
- Seasonality: The significant positive coefficient for "Summer" highlighted the strong seasonal impact on sales, indicating a need for adjustments in inventory and staffing during peak seasons.
- Event Impact: Local events significantly boosted sales, indicating opportunities for targeted promotions during those times.
Refining the Model: Iteration and Improvement
The initial regression model provided valuable insights, but Sarah understood that it was just a starting point. She explored several improvements:
- Adding More Variables: She considered including variables like competitor activity, local economic indicators, and weather patterns.
- Transforming Variables: Some variables, like advertising spend, might benefit from a logarithmic transformation to better reflect their impact on sales.
- Addressing Outliers: She carefully examined any data points that significantly deviated from the overall trend, investigating potential errors or unique circumstances.
By iteratively refining her model, Sarah achieved a more accurate and nuanced understanding of the factors influencing her sales.
Using the Model for Decision Making: Practical Applications
Sarah leveraged her improved regression model in several ways:
- Pricing Strategy: She used the model to test different pricing scenarios and assess their potential impact on sales.
- Marketing Budget Allocation: She determined the optimal level of advertising expenditure, considering the return on investment.
- Seasonal Planning: She adjusted her inventory and staffing levels based on the predicted seasonal fluctuations.
- Event Promotions: She strategically planned special promotions and offers during local events.
Beyond Linear Regression: Expanding the Data Analysis Toolkit
While linear regression provided a powerful starting point, Sarah continued her journey into data analysis, exploring other techniques:
- Time Series Analysis: To forecast future sales based on past trends.
- Customer Segmentation: To identify different customer groups and tailor marketing efforts accordingly.
- A/B Testing: To assess the effectiveness of different marketing campaigns or product variations.
Conclusion: Embracing Data for Small Business Success
Sarah's journey demonstrates the transformative power of data analysis for small business owners. Linear regression, while a relatively simple technique, provides a valuable framework for understanding the complex relationships that drive business performance. By embracing data and applying analytical tools, small businesses can make more informed decisions, reduce risk, and ultimately, achieve greater success. It's not about replacing intuition with data; it's about empowering intuition with data-driven insights. So, take the leap, gather your data, and unlock the predictive power of linear regression for your own business. Remember, continuous learning and iterative model refinement are key to maximizing the benefits of data analysis. The journey is ongoing, and the rewards are well worth the effort.
Latest Posts
Latest Posts
-
40 Days Is How Many Weeks
May 19, 2025
-
How Many Oz Is 120 Grams
May 19, 2025
-
How Many Kg In 135 Pounds
May 19, 2025
-
How Many Inches Is 42 Cm
May 19, 2025
-
800 Square Meters To Square Feet
May 19, 2025
Related Post
Thank you for visiting our website which covers about A Small Business Owner Has Created A Linear Regression . We hope the information provided has been useful to you. Feel free to contact us if you have any questions or need further assistance. See you next time and don't miss to bookmark.