A Post Hoc Test Is Warranted When
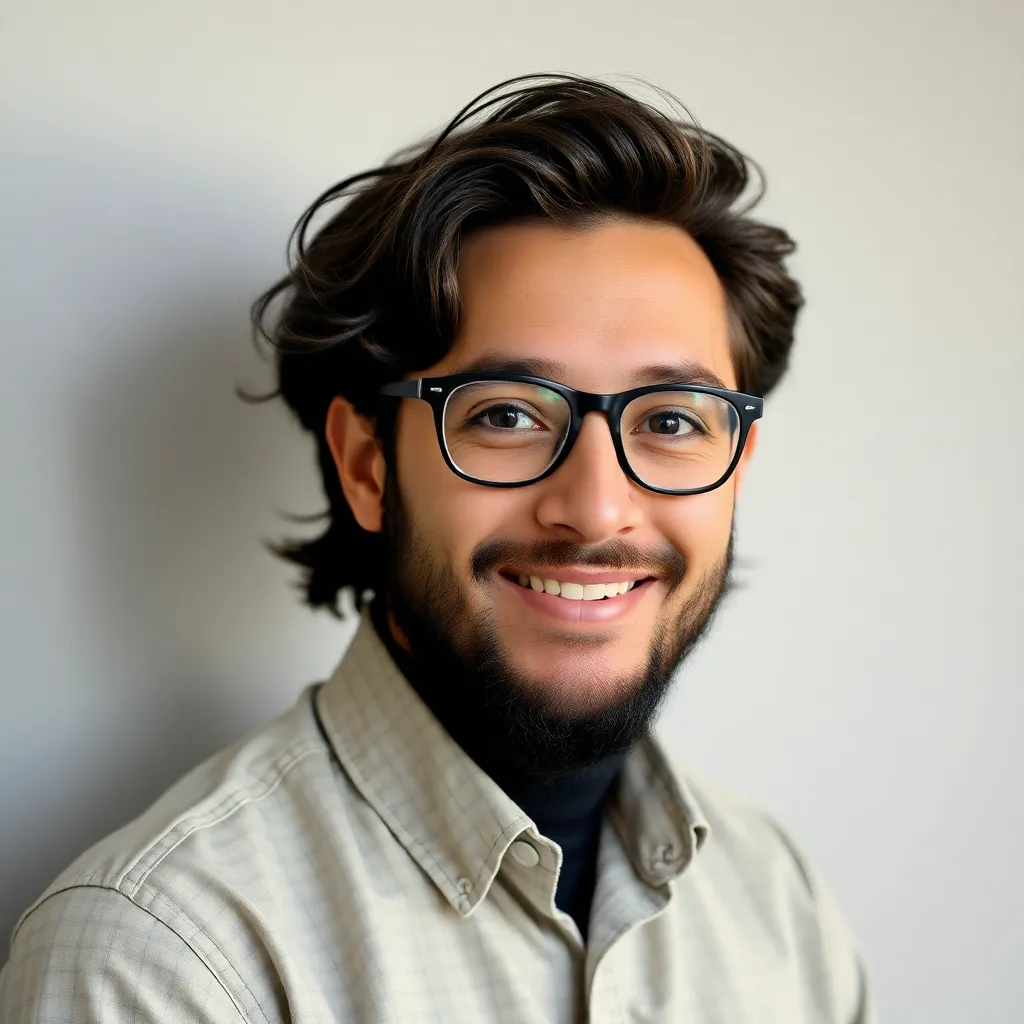
Holbox
Mar 29, 2025 · 7 min read
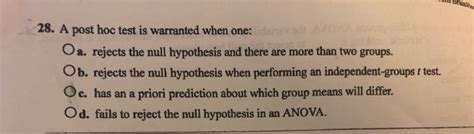
Table of Contents
- A Post Hoc Test Is Warranted When
- Table of Contents
- A Post Hoc Test is Warranted When: A Deep Dive into Statistical Significance and Multiple Comparisons
- Understanding the Need for Post Hoc Tests
- The Problem of Multiple Comparisons
- Types of Post Hoc Tests: A Comparative Overview
- 1. Bonferroni Correction
- 2. Tukey's Honestly Significant Difference (HSD) Test
- 3. Scheffé's Test
- 4. Games-Howell Test
- 5. Dunnett's Test
- Choosing the Right Post Hoc Test: A Practical Guide
- Beyond Pairwise Comparisons: Exploring Planned Contrasts
- Reporting Post Hoc Test Results
- Conclusion: The Essential Role of Post Hoc Tests
- Latest Posts
- Latest Posts
- Related Post
A Post Hoc Test is Warranted When: A Deep Dive into Statistical Significance and Multiple Comparisons
Performing statistical tests is a cornerstone of research across numerous fields. Often, researchers utilize Analysis of Variance (ANOVA) to compare means across multiple groups. However, a significant ANOVA result only indicates that at least one group mean differs significantly from the others; it doesn't pinpoint which groups differ. This is where post hoc tests come in. A post hoc test is warranted when a statistically significant result from an ANOVA (or other omnibus test) reveals an overall difference between groups, but doesn't specify where these differences lie. This article delves into the rationale behind post hoc tests, explores various types, discusses their strengths and weaknesses, and provides practical guidance on choosing the appropriate test.
Understanding the Need for Post Hoc Tests
The ANOVA test, a powerful tool for comparing means across multiple groups, provides an overall F-statistic. A significant F-statistic (typically indicated by a p-value below a pre-determined alpha level, often 0.05) suggests that there's at least one significant difference among the group means. However, this information is insufficient. Imagine comparing the effectiveness of four different teaching methods. A significant ANOVA result tells us that at least one method differs from the others, but not which method(s) are superior or inferior. This is where post hoc tests become crucial. They perform multiple pairwise comparisons, allowing us to identify the specific group differences driving the overall significant effect.
The Problem of Multiple Comparisons
The core issue behind the necessity of post hoc tests is the problem of inflated Type I error rate. Each individual comparison between two groups carries a risk of committing a Type I error – rejecting the null hypothesis (that there's no difference) when it's actually true. With multiple comparisons, the probability of making at least one Type I error accumulates. For instance, if we perform three comparisons, each with a significance level of 0.05, the overall probability of making at least one Type I error is substantially higher than 0.05. This inflation of the Type I error rate makes our results less reliable. Post hoc tests address this problem by adjusting the significance level to control the overall Type I error rate.
Types of Post Hoc Tests: A Comparative Overview
Several post hoc tests exist, each with its own assumptions, strengths, and weaknesses. The choice of a suitable test depends on several factors, including the design of the study (e.g., balanced or unbalanced design), the nature of the data (e.g., normality, homogeneity of variances), and the desired control over the Type I error rate.
1. Bonferroni Correction
The Bonferroni correction is a simple and widely used method. It adjusts the alpha level by dividing it by the number of comparisons. For example, with three comparisons and an alpha of 0.05, the adjusted alpha becomes 0.0167 (0.05/3). While straightforward, the Bonferroni correction is known to be conservative, meaning it may lead to a higher risk of Type II error (failing to reject the null hypothesis when it's false). This conservatism increases as the number of comparisons increases.
2. Tukey's Honestly Significant Difference (HSD) Test
Tukey's HSD test is a powerful and widely used post hoc test that controls the family-wise error rate (FWER). It's particularly well-suited for balanced designs (i.e., designs with equal sample sizes in each group). Tukey's HSD is less conservative than the Bonferroni correction, offering a better balance between Type I and Type II error rates. It's based on the studentized range distribution, which considers the range of the sample means.
3. Scheffé's Test
Scheffé's test is a very conservative post hoc test that controls the FWER for all possible contrasts, not just pairwise comparisons. This makes it suitable for situations where researchers might want to test more complex comparisons, such as comparing the average of two groups to a third group. However, its high conservatism makes it less powerful than other tests, increasing the likelihood of Type II errors.
4. Games-Howell Test
The Games-Howell test is a powerful option for unbalanced designs (i.e., designs with unequal sample sizes in each group) and when the assumption of homogeneity of variances is violated. Unlike some other tests, it doesn't assume equal variances across groups. This makes it robust to violations of this assumption, which is often encountered in real-world data.
5. Dunnett's Test
Dunnett's test is specifically designed for comparing multiple treatment groups to a single control group. This is common in experimental designs where researchers are interested in evaluating the effectiveness of various treatments relative to a placebo or standard treatment. It's generally more powerful than other post hoc tests in this specific scenario.
Choosing the Right Post Hoc Test: A Practical Guide
Selecting the most appropriate post hoc test involves considering several factors:
- Design: Is the design balanced (equal sample sizes) or unbalanced? Balanced designs allow for a wider range of options.
- Assumptions: Are the assumptions of normality and homogeneity of variances met? Violations of these assumptions may necessitate tests like the Games-Howell test.
- Type of Comparisons: Are you interested solely in pairwise comparisons, or do you need to test more complex contrasts? Scheffé's test provides broader coverage.
- Power vs. Conservatism: A balance needs to be struck between controlling the Type I error rate and maintaining sufficient statistical power to detect genuine differences.
Here's a simplified decision tree:
- Balanced design, assumptions met: Tukey's HSD is often a good choice.
- Unbalanced design, assumptions met: Consider using Tukey's HSD (although less powerful) or a less conservative method.
- Assumptions violated (especially homogeneity of variances): Games-Howell is a robust option.
- Comparison to a control group: Dunnett's test is appropriate.
- Complex contrasts (not just pairwise comparisons): Scheffé's test offers comprehensive control, but at the cost of power.
Beyond Pairwise Comparisons: Exploring Planned Contrasts
While post hoc tests are vital for exploring significant ANOVA results, they're often used when hypotheses aren't explicitly defined a priori. For pre-defined hypotheses, planned contrasts are a more powerful and appropriate approach. Planned contrasts allow researchers to test specific hypotheses about differences between groups before conducting the ANOVA. They offer greater statistical power than post hoc tests because they don't need to adjust for multiple comparisons in the same way. However, they should only be specified before data collection and analysis to avoid the risk of data dredging.
Reporting Post Hoc Test Results
When reporting the results of post hoc tests, it's crucial to be clear and comprehensive:
- Specify the test used: Clearly state which post hoc test was employed (e.g., Tukey's HSD, Games-Howell).
- Report adjusted p-values: Report the p-values adjusted for multiple comparisons.
- Present the results in a clear and organized manner: Tables are often the most effective way to present pairwise comparisons and their associated p-values.
- Interpret the results cautiously: Remember that statistical significance doesn't necessarily imply practical significance. Consider the effect sizes along with the p-values.
Conclusion: The Essential Role of Post Hoc Tests
Post hoc tests are an integral part of statistical analysis when dealing with multiple group comparisons. They address the critical issue of inflated Type I error rates that arise from performing multiple comparisons without adjustment. The selection of an appropriate post hoc test depends on various factors, including the study design and data characteristics. By carefully considering these factors and selecting the right test, researchers can confidently identify specific group differences, leading to more robust and reliable conclusions. Remember that choosing the right test is crucial for drawing accurate and meaningful interpretations from your data. While post hoc tests are invaluable tools, always prioritize thoughtful experimental design and the use of planned contrasts where appropriate. A well-planned study reduces the reliance on post hoc analyses and increases the overall robustness of the conclusions.
Latest Posts
Latest Posts
-
In This Problem A B C And D
Apr 01, 2025
-
People With Lighter Colored Hair Have Melanin In The
Apr 01, 2025
-
Pn Mental Health Online Practice 2023 A
Apr 01, 2025
-
What Is Cold And Comes In Cans
Apr 01, 2025
-
Which Of The Following Is A Structural Isomer To Glucose
Apr 01, 2025
Related Post
Thank you for visiting our website which covers about A Post Hoc Test Is Warranted When . We hope the information provided has been useful to you. Feel free to contact us if you have any questions or need further assistance. See you next time and don't miss to bookmark.