A Limitation Of Content Analysis Is That
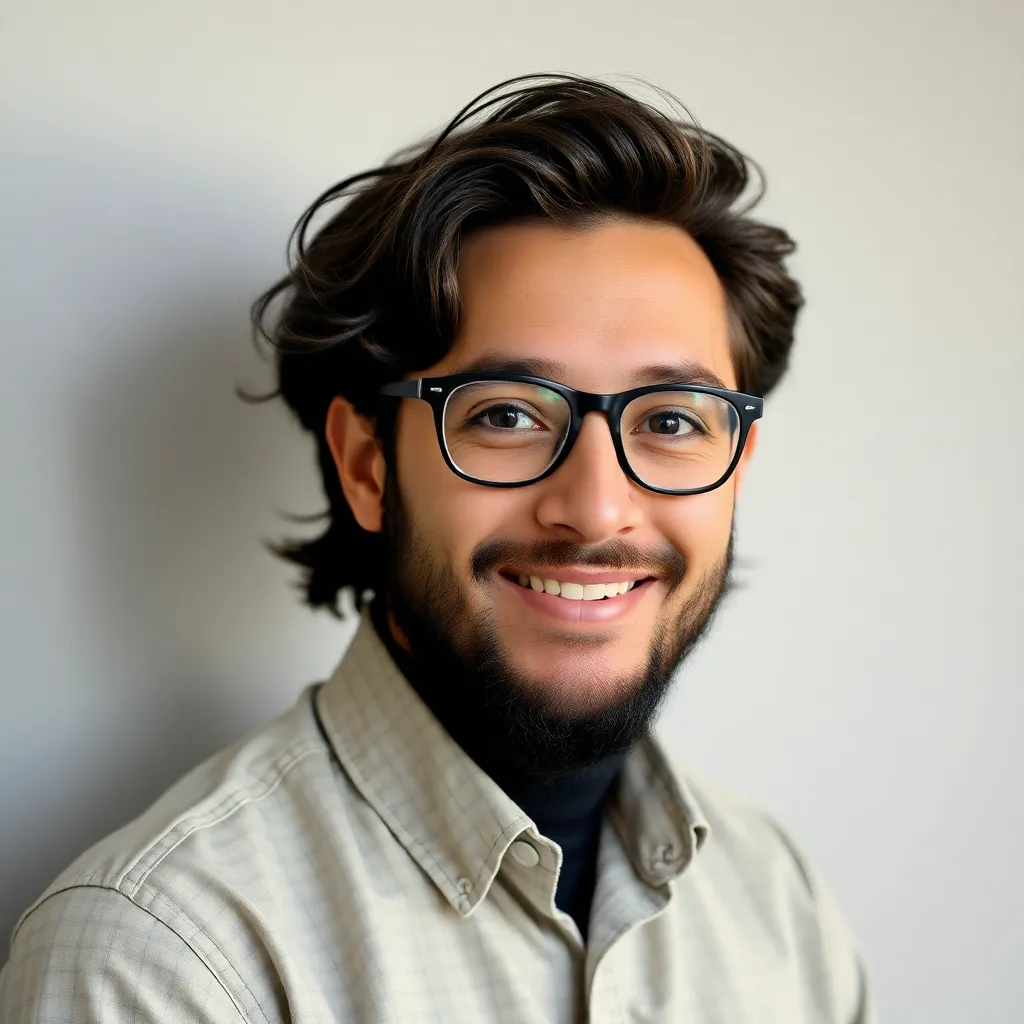
Holbox
May 10, 2025 · 7 min read
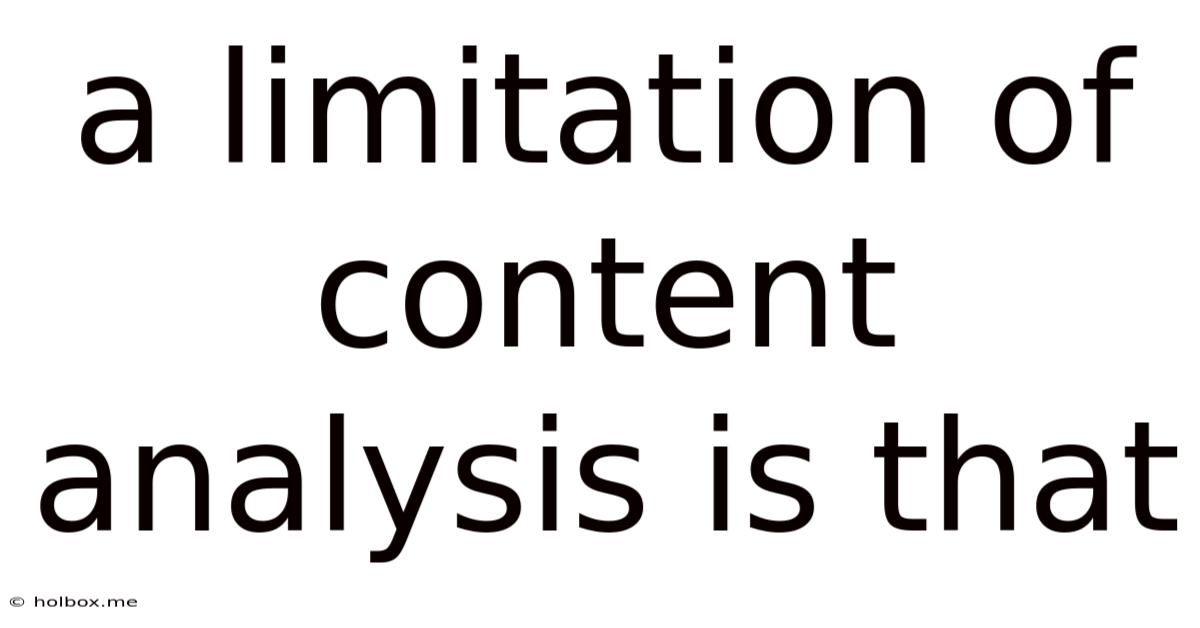
Table of Contents
- A Limitation Of Content Analysis Is That
- Table of Contents
- A Limitation of Content Analysis is That… It's Only as Good as Your Data
- The Garbage In, Garbage Out Principle
- 1. Data Representativeness: Sampling Bias and Generalizability
- 2. Data Reliability and Validity: Accuracy and Consistency
- 3. Data Quality: Contextual Factors and Ambiguity
- Beyond the Data: Limitations in the Analytical Process
- 1. Researcher Bias: Subjectivity and Interpretation
- 2. Coding Scheme Limitations: Defining Categories and Themes
- 3. The Limitations of Quantification: Reducing Richness to Numbers
- 4. Time and Resource Constraints: Feasibility and Scope
- Conclusion: Navigating the Limitations of Content Analysis
- Latest Posts
- Related Post
A Limitation of Content Analysis is That… It's Only as Good as Your Data
Content analysis, a powerful qualitative research method, allows researchers to systematically analyze and interpret textual or visual data to uncover patterns, themes, and meanings. However, despite its versatility and rich insights, content analysis is not without its limitations. One significant constraint lies in the inherent quality and nature of the data being analyzed. This article will delve into this core limitation, exploring the various aspects that affect the validity and reliability of content analysis findings, ultimately impacting the conclusions drawn from the research.
The Garbage In, Garbage Out Principle
The most fundamental limitation of content analysis is encapsulated in the adage "garbage in, garbage out." The quality of the analysis is inextricably linked to the quality of the data used. If the data is flawed, incomplete, biased, or unrepresentative, the resulting analysis will inevitably reflect these shortcomings. This section will unpack several key aspects of data quality that directly influence the limitations of content analysis.
1. Data Representativeness: Sampling Bias and Generalizability
A crucial aspect of data quality is its representativeness. If the data selected for analysis does not accurately reflect the larger population or phenomenon under investigation, the findings cannot be generalized. This can stem from various sources of sampling bias:
- Convenience Sampling: Using readily available data, such as readily accessible online forums or a limited collection of documents, can lead to a biased sample and limit the generalizability of findings. The sample may not be truly representative of the broader population of interest.
- Selection Bias: Conscious or unconscious selection of particular data points based on pre-existing assumptions or biases can skew the results. Researchers might unintentionally favour data that supports their hypothesis while ignoring contradictory evidence.
- Sampling Frame Error: The sampling frame, which defines the population from which the sample is drawn, might itself be flawed. For instance, if the study aims to analyze public opinion on a social issue but uses data solely from a specific online community, it would not reflect the broader public opinion.
Mitigation Strategies: To address this limitation, researchers must employ rigorous sampling techniques that ensure representativeness. This includes using probability sampling methods (e.g., simple random sampling, stratified sampling) whenever possible. Furthermore, transparently acknowledging the limitations of the sample and the scope of generalizability is crucial.
2. Data Reliability and Validity: Accuracy and Consistency
The reliability and validity of the data are paramount. Reliability refers to the consistency of the data, meaning that repeated measurements or analyses should yield similar results. Validity, on the other hand, refers to the accuracy of the data in measuring what it intends to measure. Several factors can compromise data reliability and validity:
- Inconsistent Data Collection Methods: Variations in data collection procedures can introduce inconsistencies. For example, different coders applying varying interpretations of coding categories can lead to unreliable data.
- Data Incompleteness or Missing Data: Gaps in the data can significantly affect the analysis. Missing data points can introduce bias and reduce the accuracy of the findings. Imputation techniques can be used, but they may introduce further limitations.
- Measurement Error: Errors in data recording or transcription can significantly impact the accuracy of the findings. Double-checking and quality control measures are essential to minimize such errors.
- Subjectivity in Interpretation: Even with clear coding schemes, different analysts might interpret the same data differently. This subjectivity is a significant source of unreliability.
Mitigation Strategies: To enhance reliability, standardized coding schemes, rigorous inter-rater reliability checks, and detailed documentation of coding procedures are crucial. Validity can be enhanced through triangulation, employing multiple data sources or methods to corroborate findings.
3. Data Quality: Contextual Factors and Ambiguity
The quality of data also depends heavily on contextual factors and the potential for ambiguity within the data itself. These factors can include:
- Ambiguous Language: Textual data can contain ambiguous language, making it challenging to assign clear meanings to specific words or phrases. Different interpretations can lead to inconsistencies in coding and analysis.
- Unclear Context: The meaning of data can be profoundly affected by the context in which it is produced. Without adequate understanding of the context, accurate interpretation can be difficult.
- Data Bias: Existing biases within the data source itself can influence the results. For instance, news articles often reflect a particular perspective, which will affect the findings if not acknowledged.
- Data Evolution: Data, particularly online content, can evolve over time. Analyzing a snapshot of data might not reflect the complete picture.
Mitigation Strategies: Careful consideration of contextual factors, development of robust coding schemes that address ambiguity, and awareness of potential biases within the data source are vital for mitigating these limitations. Researchers should clearly document the limitations imposed by these factors in their analysis.
Beyond the Data: Limitations in the Analytical Process
Even with high-quality data, other limitations inherent in the content analysis process itself can impact the reliability and validity of the findings:
1. Researcher Bias: Subjectivity and Interpretation
The researcher's own biases, beliefs, and interpretations can unintentionally influence the analysis. The process of selecting data, developing coding schemes, and interpreting results is not entirely objective. Unconscious biases can lead to selective attention to certain aspects of the data while overlooking others.
Mitigation Strategies: Researchers should be mindful of their own potential biases and strive for reflexivity. Employing multiple researchers to independently analyze the data and comparing results can help to mitigate this limitation. Transparency in the research methods and a critical self-evaluation of potential biases are also essential.
2. Coding Scheme Limitations: Defining Categories and Themes
The development of a robust and comprehensive coding scheme is crucial for a successful content analysis. However, the process of categorizing and defining themes can be subjective and potentially limited.
- Overlapping Categories: Poorly defined categories can lead to overlap and ambiguity in coding, affecting the accuracy of the results.
- Exhaustive Categories: The categories chosen should be sufficiently comprehensive to encompass all relevant aspects of the data. Insufficient categories can lead to important information being overlooked.
- Mutually Exclusive Categories: Categories should be mutually exclusive; data points should only fall into one category to avoid double-counting.
Mitigation Strategies: The creation of a well-defined and clearly documented coding scheme is paramount. Pilot testing the coding scheme and seeking feedback from other researchers can help to identify and address potential limitations. Inter-rater reliability checks are crucial to ensure consistency in coding.
3. The Limitations of Quantification: Reducing Richness to Numbers
Content analysis often involves quantifying qualitative data. While quantification provides a structured way to analyze large datasets, it risks reducing the richness and nuance of the data to simple numbers. Important contextual information might be lost in the process of quantification. The numerical representation might not fully capture the complexities of the data.
Mitigation Strategies: Researchers should strive for a balanced approach, combining quantitative analysis with qualitative interpretations to capture both the breadth and depth of the data. Presenting both numerical summaries and illustrative quotes or examples can provide a richer and more nuanced understanding of the findings.
4. Time and Resource Constraints: Feasibility and Scope
Conducting a thorough content analysis can be time-consuming and resource-intensive. The sheer volume of data to be analyzed, the complexity of the coding scheme, and the need for rigorous quality control can limit the feasibility and scope of the research.
Mitigation Strategies: Careful planning, clear research objectives, and the use of appropriate software tools can help to manage time and resource constraints. Researchers might choose to focus on a smaller, more manageable dataset to ensure the thoroughness and quality of the analysis.
Conclusion: Navigating the Limitations of Content Analysis
Content analysis offers a powerful approach to understanding complex textual and visual data. However, it is crucial to acknowledge and address the limitations inherent in the method. The quality of the data, the analytical process, and the potential for bias all influence the validity and reliability of the findings. By employing rigorous data collection techniques, developing robust coding schemes, maintaining transparency in the research process, and being mindful of potential biases, researchers can mitigate these limitations and draw meaningful conclusions from their content analyses. The key is a critical and reflective approach that acknowledges the inherent complexities of the research process and strives to provide transparent and robust findings. Failing to address these limitations can lead to misleading or inaccurate interpretations of the data, undermining the credibility and value of the research.
Latest Posts
Related Post
Thank you for visiting our website which covers about A Limitation Of Content Analysis Is That . We hope the information provided has been useful to you. Feel free to contact us if you have any questions or need further assistance. See you next time and don't miss to bookmark.