A Histogram That Is Positively Skewed Is Also Called
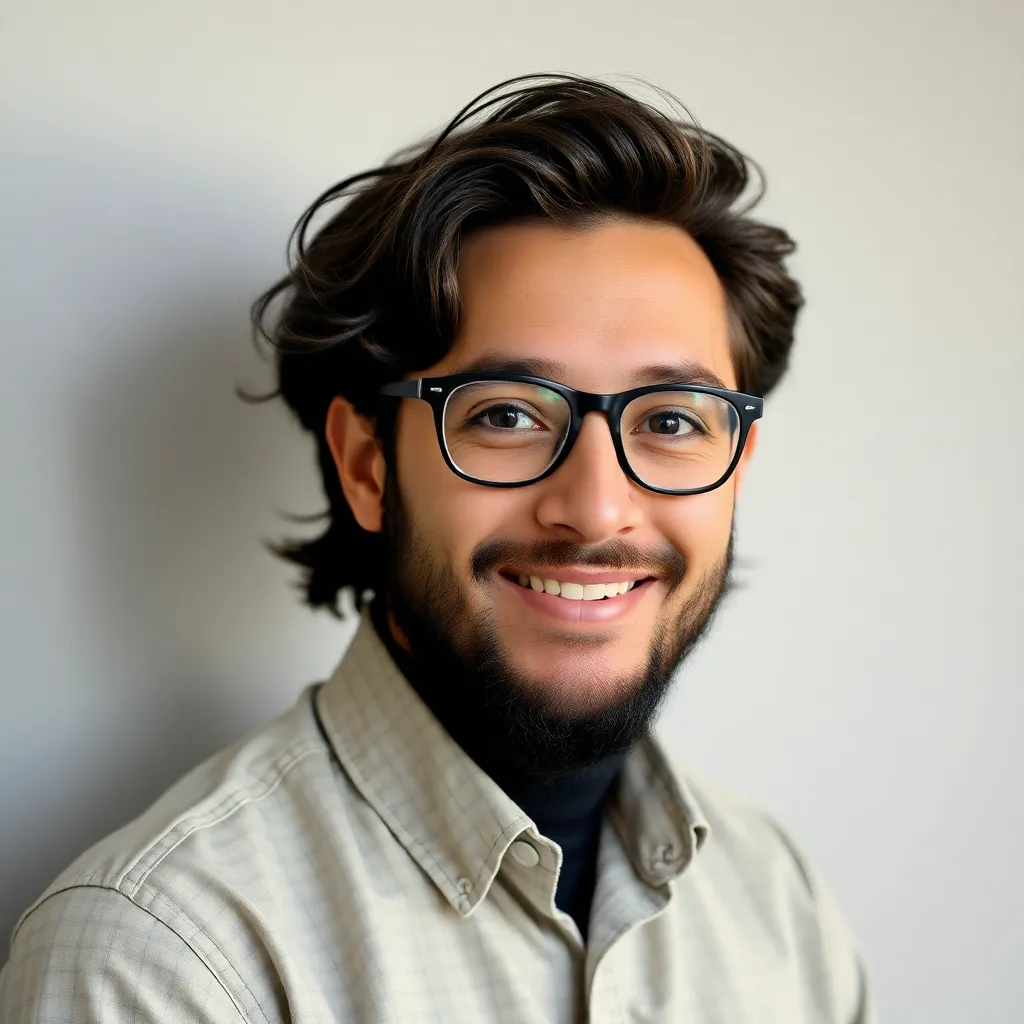
Holbox
May 11, 2025 · 6 min read
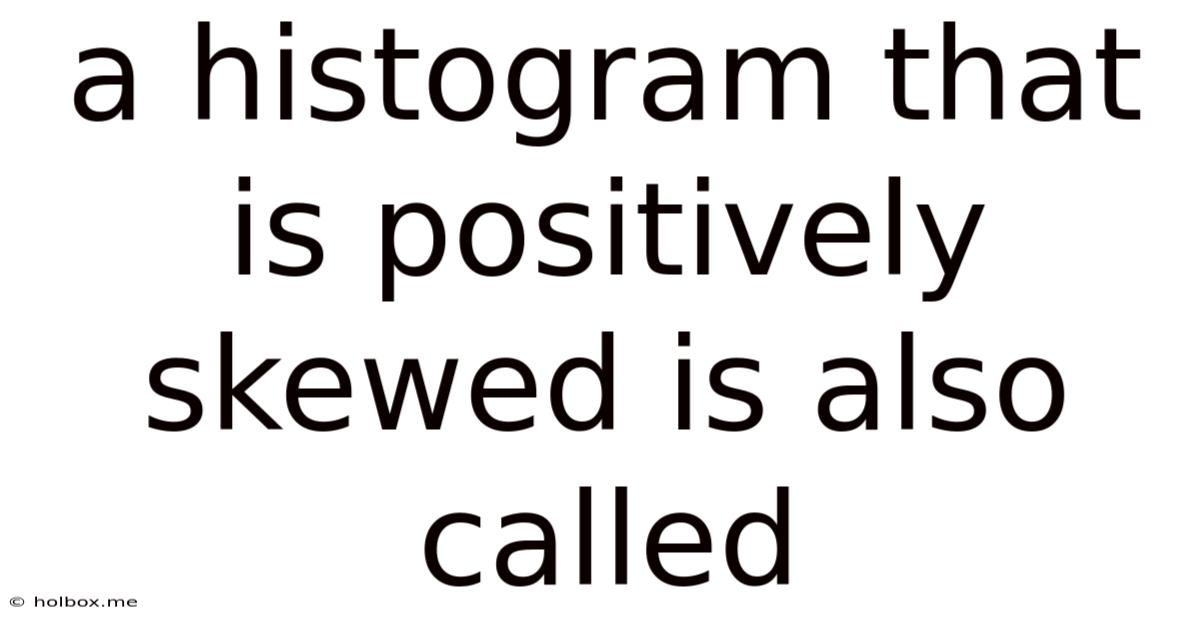
Table of Contents
- A Histogram That Is Positively Skewed Is Also Called
- Table of Contents
- A Histogram That is Positively Skewed is Also Called: Understanding Skewness and its Implications
- Understanding Skewness: The Shape of Data Distributions
- Positively Skewed Histograms: Alternative Names and Descriptions
- Interpreting Positively Skewed Data: Real-World Examples
- Consequences of Ignoring Positive Skewness: Statistical Analysis and Interpretation
- Handling Positively Skewed Data: Transformations and Alternatives
- Conclusion: The Importance of Understanding and Addressing Positive Skewness
- Latest Posts
- Related Post
A Histogram That is Positively Skewed is Also Called: Understanding Skewness and its Implications
A histogram, a powerful visual tool in statistics, provides a graphical representation of the distribution of numerical data. Its bars illustrate the frequency or relative frequency of data points falling within specific ranges or bins. When the distribution isn't symmetrical, we observe skewness, a crucial characteristic influencing data interpretation and statistical analysis. This article delves into the concept of positive skewness, exploring what it means, alternative names used to describe it, and its significance across various fields.
Understanding Skewness: The Shape of Data Distributions
Skewness describes the asymmetry of a probability distribution. A perfectly symmetrical distribution, like a normal distribution (bell curve), has a skewness of zero. However, real-world data rarely exhibits perfect symmetry. Skewness can be positive (right-skewed), negative (left-skewed), or even zero, indicating symmetry.
Visualizing Skewness: Imagine a histogram. If the tail of the distribution stretches longer to the right (towards higher values), it is positively skewed. Conversely, if the tail extends longer to the left (towards lower values), it's negatively skewed.
Mathematical Definition of Skewness: While visual inspection is helpful, skewness can be quantified using statistical measures. The most common measure is Pearson's moment coefficient of skewness, which involves calculations using the mean, median, and standard deviation of the data. A positive value indicates positive skewness, a negative value indicates negative skewness, and a value close to zero suggests symmetry.
Positively Skewed Histograms: Alternative Names and Descriptions
A histogram exhibiting positive skewness is characterized by a long right tail. This implies that a larger proportion of data points are concentrated on the lower end of the distribution, while a smaller number of data points are scattered at the higher end. This creates the visually distinct rightward skew. This type of distribution is also frequently referred to by other names, including:
-
Right-skewed histogram: This is the most straightforward and commonly used alternative. The term directly reflects the direction of the tail's extension.
-
Right-tailed histogram: Similar to "right-skewed," this emphasizes the presence of a long tail extending to the right.
-
Asymmetrical histogram (with a right skew): This highlights the lack of symmetry, specifically pointing towards the rightward asymmetry.
-
Positively skewed frequency distribution: This term uses a more formal statistical terminology and precisely describes the nature of the distribution.
-
Long-tailed histogram (to the right): This emphasizes the presence of outliers or extreme values that pull the tail to the right.
All of these terms accurately describe a histogram with a positive skew, and the choice of which term to use often depends on the context and the audience. In academic settings, the terms "positively skewed" or "right-skewed" are generally preferred for their clarity and precision. In more informal settings, "right-tailed" might be used.
Interpreting Positively Skewed Data: Real-World Examples
Understanding positive skewness goes beyond mere visual identification. It provides valuable insights into the nature of the data and the underlying process generating it. Let's examine some illustrative examples:
-
Income Distribution: Income data often exhibits positive skewness. The majority of individuals earn within a certain range, while a small percentage of high earners significantly inflate the mean. This creates a long tail extending towards higher income levels.
-
Real Estate Prices: Similar to income, housing prices in a given area tend to be positively skewed. Most houses fall within a specific price bracket, but the presence of luxury properties pulls the tail to the right, leading to a positive skew.
-
Test Scores: In an easy examination where most students perform well, but a few students struggle, the distribution of scores could show a positive skew. The majority of scores would be clustered on the higher end, with a few low scores forming the tail to the left.
-
Customer Spending: In businesses analyzing customer purchase amounts, positively skewed distributions might indicate that most customers make relatively small purchases, but a few big-spending customers inflate the average.
-
Waiting Times: Waiting times in a system might have a positive skew if a majority of customers experience relatively short wait times, but occasional significant delays lead to longer waiting periods.
The implications of positive skewness in these examples are significant. Simply using the mean as a representative measure can be misleading. The mean will be inflated by the high values in the right tail, not accurately reflecting the typical values. In these cases, the median (the middle value when data is ordered) or other robust measures of central tendency are more appropriate.
Consequences of Ignoring Positive Skewness: Statistical Analysis and Interpretation
Ignoring positive skewness can lead to several problems in statistical analysis and interpretation:
-
Misleading Mean: As mentioned earlier, the mean is highly sensitive to outliers in positively skewed data. It will be larger than the median, providing a distorted representation of the typical value.
-
Inaccurate Statistical Tests: Many statistical tests assume a normal distribution. Applying these tests to positively skewed data without transformation can yield unreliable results.
-
Inappropriate Model Selection: Choosing statistical models unsuitable for skewed data can lead to inaccurate predictions and inferences.
-
Poor Decision Making: Based on flawed analysis, business or policy decisions might be made that are not aligned with the actual data.
Handling Positively Skewed Data: Transformations and Alternatives
Several strategies can mitigate the effects of positive skewness:
-
Data Transformation: Applying mathematical transformations such as logarithmic transformations (log), square root transformations, or Box-Cox transformations can often normalize skewed data, making it more suitable for standard statistical methods.
-
Non-parametric methods: Non-parametric tests do not assume a specific distribution, making them suitable for skewed data. These methods, such as the Wilcoxon rank-sum test or the Mann-Whitney U test, compare ranks rather than raw data values.
-
Robust Statistical Measures: Using the median instead of the mean and using measures of dispersion like the interquartile range instead of the standard deviation is less sensitive to the influence of outliers and provides a more accurate representation.
-
Visualization Techniques: Alongside histograms, box plots are also useful to visualize the distribution and identify outliers in positively skewed data.
The best approach for handling positive skewness depends on the specific dataset and the goals of the analysis.
Conclusion: The Importance of Understanding and Addressing Positive Skewness
A positively skewed histogram, also known as a right-skewed, right-tailed, or positively skewed frequency distribution, signifies a specific pattern in data, often characterized by a long tail extending to the right. This pattern often indicates the presence of outliers or extreme values that disproportionately influence traditional statistical measures like the mean. Understanding this skewness is crucial for appropriate data interpretation and statistical analysis. Failure to account for positive skewness can lead to misleading conclusions, inaccurate predictions, and ultimately, poor decision-making. Through various techniques, such as data transformations, non-parametric methods, and robust statistical measures, the challenges posed by positively skewed data can be effectively addressed, facilitating a more accurate and insightful analysis. Remember that choosing the best approach requires careful consideration of the specific characteristics of your dataset and your research objectives.
Latest Posts
Related Post
Thank you for visiting our website which covers about A Histogram That Is Positively Skewed Is Also Called . We hope the information provided has been useful to you. Feel free to contact us if you have any questions or need further assistance. See you next time and don't miss to bookmark.