A Bias Of -10 Means Your Method Is _____ Forecasting
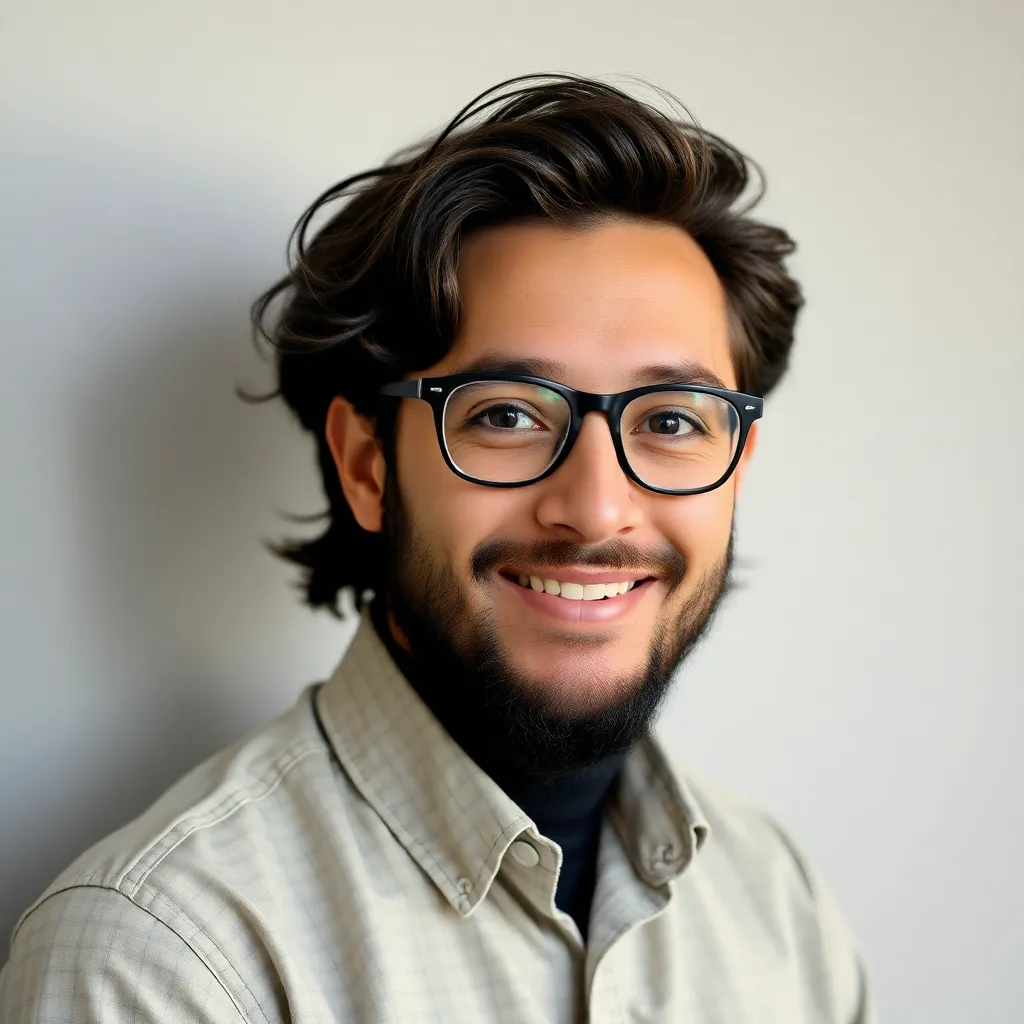
Holbox
Apr 02, 2025 · 5 min read
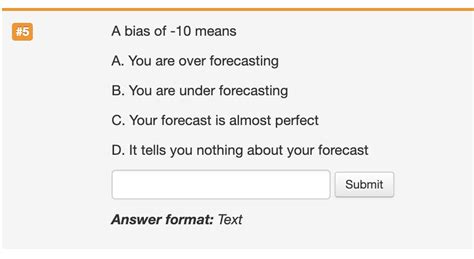
Table of Contents
- A Bias Of -10 Means Your Method Is _____ Forecasting
- Table of Contents
- A Bias of -10 Means Your Method is Underforecasting: Understanding and Mitigating Forecasting Errors
- What is Forecasting Bias?
- Understanding a -10 Bias: The Implications of Underforecasting
- Consequences of Underforecasting:
- Identifying the Sources of Underforecasting Bias
- 1. Data Issues:
- 2. Model Limitations:
- 3. External Factors:
- Mitigating Underforecasting Bias: Practical Strategies
- 1. Data Enhancement:
- 2. Model Refinement:
- 3. Incorporating External Factors:
- 4. Monitoring and Evaluation:
- Conclusion: The Importance of Accurate Forecasting
- Latest Posts
- Latest Posts
- Related Post
A Bias of -10 Means Your Method is Underforecasting: Understanding and Mitigating Forecasting Errors
Forecasting is a crucial element in various fields, from business and finance to weather prediction and public health. Accurate forecasting allows for proactive decision-making, resource allocation, and risk management. However, forecasting methods are rarely perfect, and biases often creep into the predictions. Understanding these biases is key to improving forecasting accuracy. This article delves into the implications of a -10 bias in forecasting, explaining why it signifies underforecasting and outlining strategies to mitigate this error.
What is Forecasting Bias?
Forecasting bias refers to a systematic error in a forecasting method that consistently overestimates or underestimates the actual value. Unlike random errors, which fluctuate unpredictably, bias represents a persistent deviation from the true value. Several factors can contribute to forecasting bias, including:
- Data limitations: Incomplete, inaccurate, or outdated data can lead to biased predictions.
- Model misspecification: Using an inappropriate model for the data can introduce bias.
- Human error: Subjectivity in judgment, assumptions, and data interpretation can influence forecasts.
- Ignoring external factors: Failing to account for relevant external factors impacting the variable being forecast.
A positive bias indicates consistent overestimation, while a negative bias suggests consistent underestimation. A bias of -10, therefore, means that the forecasting method consistently underestimates the actual value by 10 units. The units depend on the variable being forecasted (e.g., sales in dollars, temperature in degrees Celsius, number of customers).
Understanding a -10 Bias: The Implications of Underforecasting
A bias of -10 in a forecasting model signifies that your method is systematically underpredicting the true value. This consistent underestimation can have serious consequences depending on the context.
Consequences of Underforecasting:
- Insufficient resource allocation: In business, underforecasting demand could lead to stockouts, lost sales opportunities, and dissatisfied customers. Insufficient staffing levels due to underestimation of workload can also negatively impact productivity and service delivery.
- Missed opportunities: Underestimating market growth or potential profits can cause businesses to miss crucial investment opportunities or expansion possibilities.
- Financial losses: Underforecasting revenue can lead to inaccurate budgeting and financial planning, potentially resulting in significant financial losses.
- Operational inefficiencies: Underestimating resource needs can result in operational bottlenecks and delays, hindering overall efficiency.
- Reputational damage: Consistent underperformance in forecasts can erode trust among stakeholders, clients, and investors.
- Safety concerns: In fields like weather forecasting or public health, underestimation can have severe safety implications. Underestimating the severity of a storm or a pandemic can lead to inadequate preparedness and potentially catastrophic consequences.
Identifying the Sources of Underforecasting Bias
Pinpointing the root cause of the -10 bias is crucial for effective mitigation. Several potential sources should be investigated:
1. Data Issues:
- Data quality: Assess the accuracy, completeness, and reliability of the historical data used for model training. Inaccurate or missing data points can lead to significant biases. Consider data cleaning and imputation techniques to handle missing or erroneous values.
- Data selection bias: Ensure the data used is representative of the future conditions being forecast. Using data from a specific period that does not accurately reflect the current trend can lead to inaccurate predictions.
- Outliers: Extreme data points can disproportionately influence model fitting and introduce bias. Examine outliers carefully to determine whether they represent genuine values or errors requiring removal or adjustment.
2. Model Limitations:
- Model specification: The chosen forecasting model may be inappropriate for the data characteristics. Consider alternative models such as ARIMA, exponential smoothing, or machine learning techniques. Explore model diagnostics to assess the model’s adequacy and identify areas of weakness.
- Parameter estimation: Improper parameter estimation can result in biased forecasts. Employ robust estimation techniques and validate the model parameters through appropriate statistical tests.
- Lack of explanatory variables: The model might be missing crucial explanatory variables that significantly influence the variable being forecast. Consider including additional relevant predictors to enhance predictive power and reduce bias.
3. External Factors:
- Seasonality and trend: Failing to account for seasonal fluctuations or underlying trends in the data can lead to consistent underestimation. Implement appropriate seasonal adjustment techniques or incorporate trend components into the forecasting model.
- Unforeseen events: Unexpected events like economic shocks, natural disasters, or technological breakthroughs can significantly affect forecasts. Consider incorporating scenario planning or incorporating uncertainty quantification into your forecasting process.
- Changes in market dynamics: Shifts in consumer behavior, competition, or technological advancements can impact forecasts. Regularly review and update your forecasting models to reflect evolving market conditions.
Mitigating Underforecasting Bias: Practical Strategies
Addressing a -10 bias requires a multi-pronged approach focusing on data quality, model improvement, and incorporating external factors. Here are some practical strategies:
1. Data Enhancement:
- Data cleaning: Thoroughly clean the dataset to remove inconsistencies, errors, and outliers.
- Data augmentation: If data is limited, consider augmenting the dataset using techniques like bootstrapping or synthetic data generation.
- Feature engineering: Create new variables from existing data that may improve predictive power.
2. Model Refinement:
- Model selection: Evaluate different forecasting models and choose the one that best fits the data and accurately captures the underlying patterns.
- Parameter tuning: Optimize the model parameters using techniques like cross-validation to minimize bias and improve accuracy.
- Ensemble methods: Combine multiple forecasting models to improve overall accuracy and reduce the impact of individual model biases.
3. Incorporating External Factors:
- Qualitative information: Incorporate expert opinions, market research, and other qualitative information into the forecasting process.
- Scenario planning: Develop multiple scenarios to account for different potential outcomes and uncertainties.
- Regular model updates: Continuously update the forecasting model to reflect changes in the environment and new data availability.
4. Monitoring and Evaluation:
- Bias monitoring: Regularly monitor the forecasting bias to track progress and identify any persistent issues.
- Error analysis: Conduct thorough error analysis to understand the sources of forecast errors and guide future improvements.
- Performance evaluation: Use appropriate metrics such as Mean Absolute Error (MAE), Root Mean Squared Error (RMSE), and Mean Absolute Percentage Error (MAPE) to assess the forecast accuracy.
Conclusion: The Importance of Accurate Forecasting
A forecasting bias of -10 indicates a significant underestimation problem that can lead to several negative consequences. Addressing this bias requires a thorough investigation into the data, the forecasting model, and external factors. By implementing the strategies outlined in this article, organizations and individuals can improve their forecasting accuracy, make more informed decisions, and mitigate potential risks associated with inaccurate predictions. Remember, continuous monitoring, evaluation, and refinement are crucial for maintaining accurate and reliable forecasts over time. Investing in robust forecasting methodologies is an investment in informed decision-making and a sustainable future. The pursuit of accurate forecasting is an ongoing process, requiring constant vigilance and adaptation to ever-changing conditions.
Latest Posts
Latest Posts
-
Which Is A Physiological Description Rather Than An Anatomical One
Apr 05, 2025
-
Complete The Table Classifying Different Types Of Anemia
Apr 05, 2025
-
Physical Exam And Health Assessment Jarvis
Apr 05, 2025
-
Policies Based On Abc Analysis Might Include Investing
Apr 05, 2025
-
When A Person Blinks She Is Using Her
Apr 05, 2025
Related Post
Thank you for visiting our website which covers about A Bias Of -10 Means Your Method Is _____ Forecasting . We hope the information provided has been useful to you. Feel free to contact us if you have any questions or need further assistance. See you next time and don't miss to bookmark.